A successful case of reducing ‘false-positives’ by applying a deep learning model to detect ‘type 1 errors’
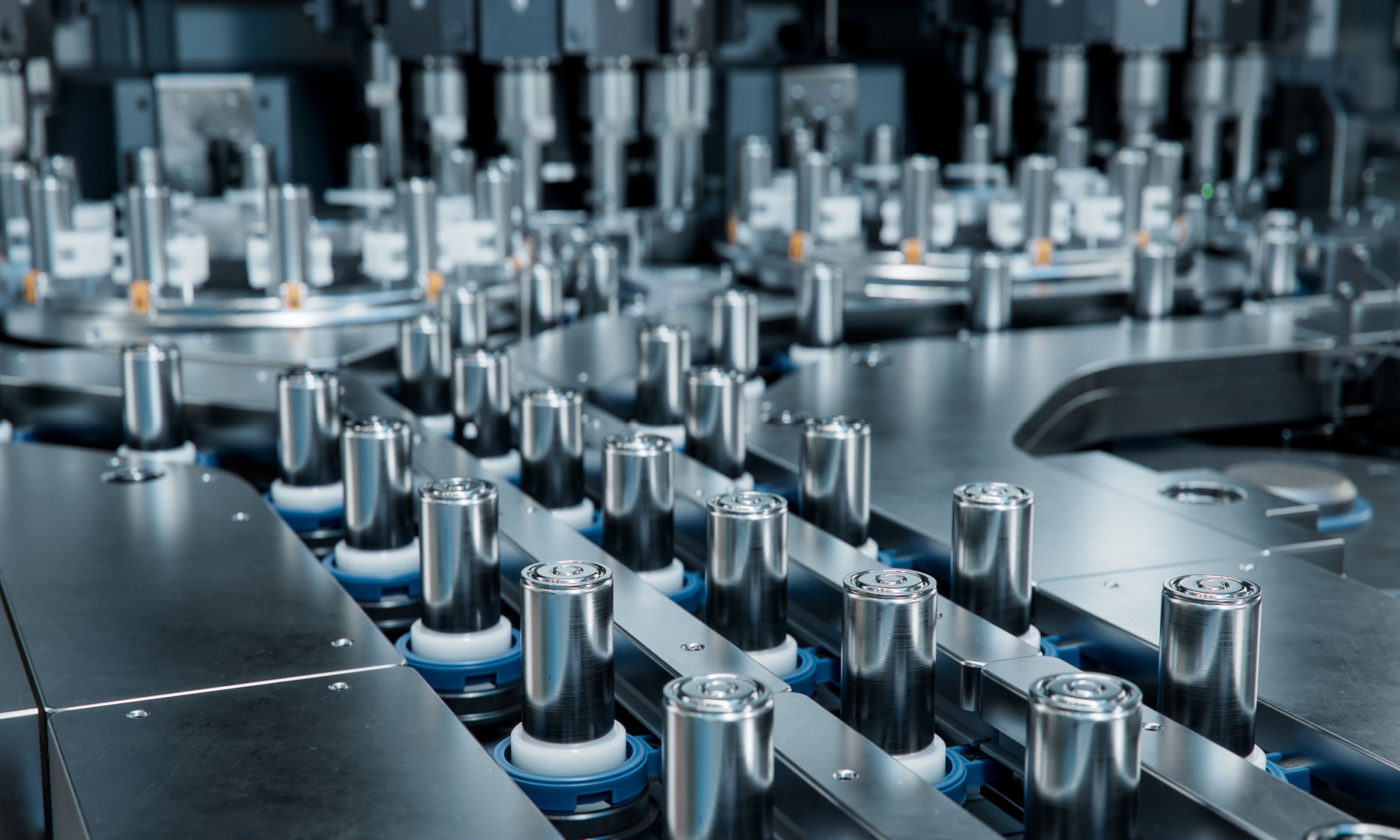
Challenge
- If the test standard is set too sensitively to achieve 0% ‘false-negative’, ‘false-positive (type 1 error)’ increases.
- Inefficiency due to random sampling and visual inspection to detect false-positives
Approach
- LISA’s type 1 error inference model learns not only the inspector’s ‘Region of interest’ but also the entire image region.
- Infer the probability of a false-positive case due to another problem rather than a defect in the actual product
- Inference results can be continuously monitored and statistical analysis performed through the Data CAMP dashboard.
Result
- Save labor costs and time by inspecting products with a high probability of Type 1 errors first
- Fast model optimization by labeling and retraining false-positive cases as normal
Full Story
Absence of false-positive re-inspection and history management tools to stabilize quality inspection
With the rise of smart factories and industrial automation, inspection automation processes are rapidly being adopted across various manufacturing sites. However, the reliability of inspection software or AI models often poses challenges.
Firstly, undetected defects, known as ‘false-negative’, are critical, especially when defective products can lead to large-scale recalls or casualties. Therefore, achieving 0% undetected defects is the primary goal when implementing inspection automation.
However, this increases the likelihood of false positives. Rule-based inspection software can be overly sensitive, detecting non-defective items as defective. Statistically, this is a type 1 error (false positive), whereas undetected defects are type 2 errors.
- False positives refer to instances where a product is normal, but quality tests indicate defects.
- Conversely, false negatives occur when defective products are mistakenly classified as normal.
Managing false-positive history is crucial, particularly when setting up new factories or product lines, to stabilize inspection processes swiftly.
The current process requires manual inspection, where all products marked defective are rechecked, often recorded manually in Excel software, which is time-consuming and labor-intensive.
Company E, a secondary battery manufacturer, sought to address these issues using deep learning.
Infer False-Positive Probability by Learning Entire Image Area
Company E, a secondary battery production company, was collecting and analyzing captured images and quality inspection data produced by an inspection machine using Data CAMP, AHHA Labs’ data integration solution. Additionally, LISA was linked to manage false-positive history.
LISA is AHHA Labs’ industrial AI solution and includes a deep learning model (Anomaly Detection) that infers type 1 errors. Type 1 error inference works as follows:
The inspector examines specific areas of the product image, called the ROI (Region of Interest). However, if the inspection software sets the ROI incorrectly, a normal product may be mistakenly judged as defective (NG), resulting in a false positive.
LISA’s Anomaly Detector, however, learns from the entire image area, not just the ROI. This allows it to infer the likelihood of a false-positive case due to issues other than an actual product defect.
The results inferred by LISA can be continuously monitored and analyzed statistically through the Data CAMP dashboard.
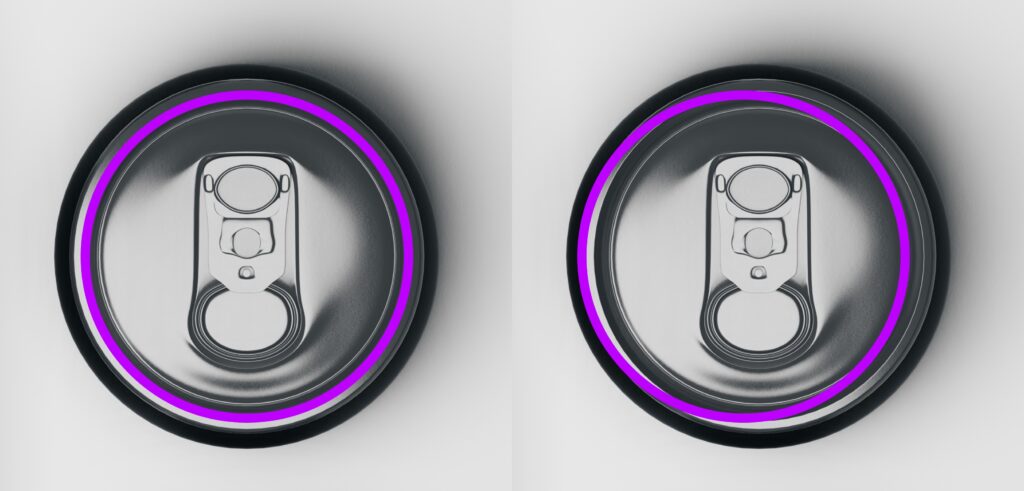
The inspector examines a specific area (purple circle) in an image of the product. However, if an error occurs in the inspection software and the ROI (Region of Interest) is set incorrectly, a normal product may be incorrectly judged as defective (NG) (right photo). This is a false positive (type 1 error). Image Credit: AHHA Labs.
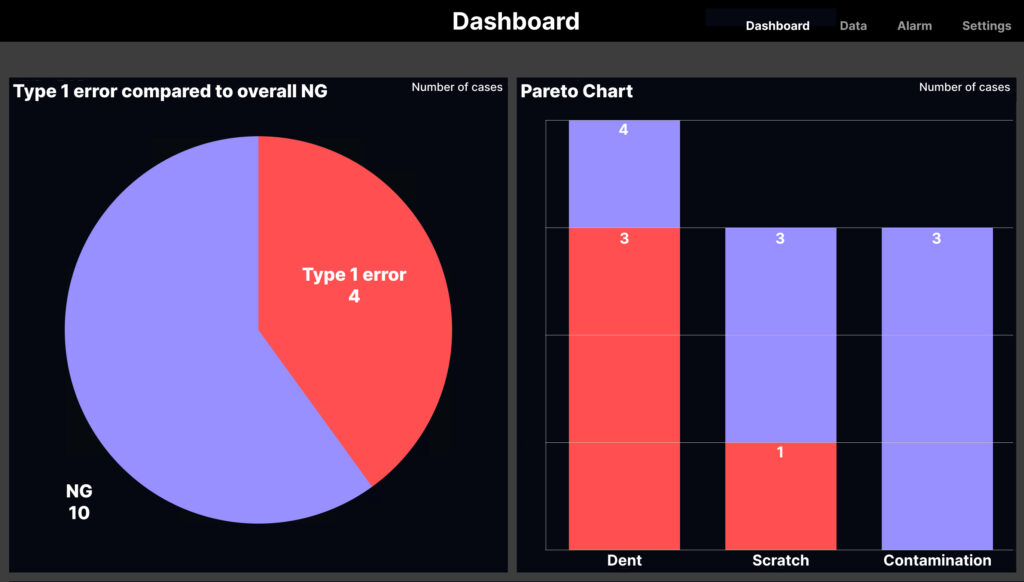
Example image of a dashboard monitoring type 1 errors (false-positives). The pie chart on the left shows the ratio of the quantity (red) that LISA judged to be highly likely to be a type 1 error compared to the total quantity that the tester judged to be NG. Image Credit: AHHA Labs
Result
- Save labor costs and time by inspecting products with a high probability of Type 1 errors first
- Fast model optimization by labeling and retraining false-positive cases as normal
Until now, they detected false-positive through random sampling and visual inspection. Now, using AHHA Labs’ type 1 error discrimination model, they can first filter out samples likely to be false-positive and review them.
Compared to before, they reduced re-inspection time and increased the accuracy of detecting false-positives.
Additionally, quickly feeding back to inspection software allows the inspection process to be optimized in a shorter period. As production and inspection progress, they can easily manage history, including the extent of false-positive and changing trends.
AI Inspection with 86% Cost Reduction Using On-Device AI
Challenge Defects caused by external contaminants are unpredictable in shape,...
Transforming Battery Manufacturing with AI Inspection & Data Automation
From Manual Inspections to Smart Factories: The Evolution of Battery...
A 3-Step AI Inspection Pipeline for Rubber Sheet Defects: Automating Detection, Classification, and Location Analysis
Challenge In the tire manufacturing process, rubber sheets produced during...
Boosting Control Efficiency of Battery Assembly Lines by 300% Through a Remote Monitoring System
Challenge The assembly lines for EV batteries are massive and highly complex,...
Detecting Inspection Data Drift Caused by Material Changes: A Case Study on the Data Quality Index (DQI) Model
Challenge In manufacturing, consistently capturing high-quality...