LISA
Sustainability of AI system operation
LISA continuously employs the latest research methodologies for data generation and inspection,
achieving industry-leading speed and performance at multiple global manufacturing sites
LISA continuously employs the latest research methodologies for data generation and inspection, achieving industry-leading speed and performance at multiple global manufacturing sites
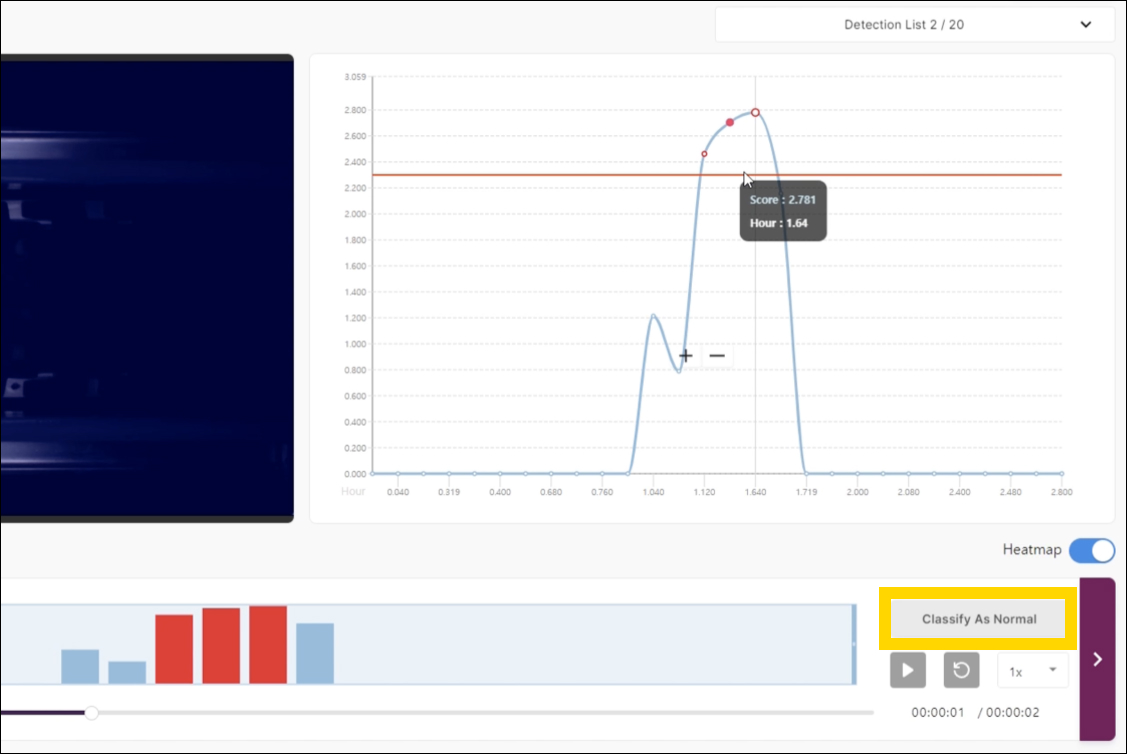
False-positive data recommendation and automatic labeling/retraining
Reducing false positives is crucial in product quality control. Excessive false positives increase costs and decrease production efficiency, so minimizing them directly lowers the operating costs of automated quality inspections. LISA employs a deep learning model(i.e., Anomaly Detector) pipeline to automatically identify and recommend data deemed false-positive (type 1 error) based on the existing rule-based inspection results at the manufacturing site.
Additionally, clicking the ‘Classify as Normal’ button while reviewing the Anomaly Detection results instantly relabels the data as normal. This change is immediately reflected in the dataset for use in model retraining, enabling you to optimize your model quickly.
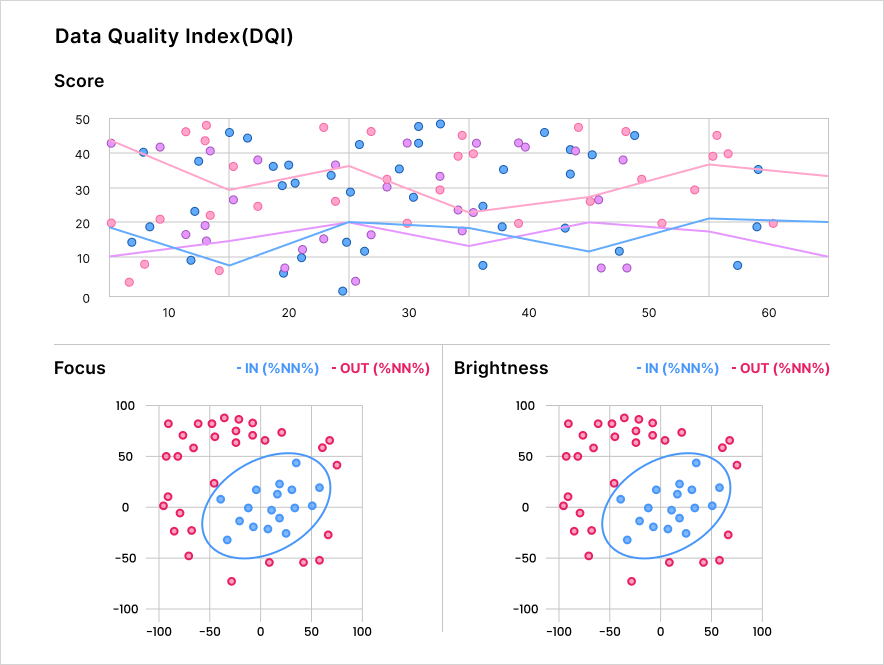
Monitor data and model drift
Monitoring data drift and model drift is essential for performance management and continuous optimization in machine learning systems. If you don’t properly manage drift, your model’s predictive accuracy can deteriorate over time, which can ultimately have a negative impact on your business.
‘Data Quality Index (DQI)’ is AHHA Labs’ unique deep learning model that monitors drift in input data. If a new input image significantly differs in focus or brightness from the reference image, making it unsuitable for defect determination, a warning alarm is immediately triggered. Users can click on the outlier data displayed on the dashboard to view images classified as abnormal and take necessary actions, such as changing camera settings or replacing old lighting.
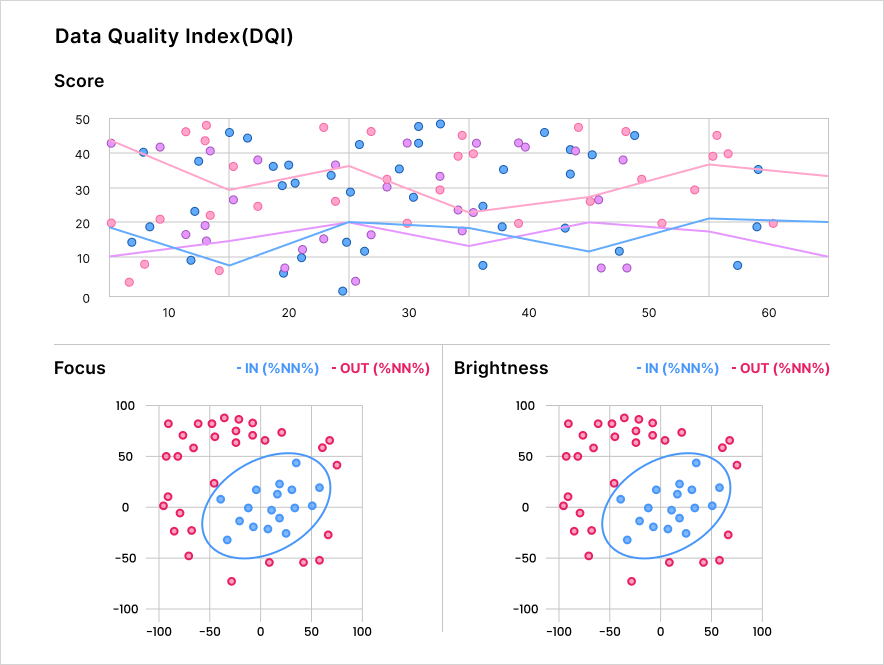
Achieve Your Business Goals
with a Convenient MLOps Platform
Achieve Your Business Goals with a Convenient MLOps Platform
A successful case of reducing ‘false-positives’ by applying a deep learning model to detect ‘type 1 errors’
Challenge If the test standard is set too sensitively to achieve 0%...
Success case of monitoring ‘data drift’ and performing predictive maintenance with a data quality index (DQI) model
Challenge Consistent optical images must be taken at all times to...
Configuring an AI model pipeline to detect robotic grasping anomalies in real time
Challenge Robot drops battery, causing downtime Difficulty utilizing...
Next Step →
With LISA, dramatically increase productivity and quality, considering both quick introduction and sustainable operation.