LISA
Easy to use UI for domain expert and citizen scientist
LISA offers detailed metrics and visual heatmaps of model performance,
providing insights into AI model development and utilization.
LISA offers detailed metrics and visual heatmaps of model performance, providing insights into AI model development and utilization.
Detailed metrics on model performance
LISA automatically calculates a various performance metrics and offers a detailed confusion matrix of hits, misses, and overfits, giving an intuitive view of a model’s predictive performance. This enables users to comprehend the model’s overall performance and compare different models to quickly identify and apply the best one for a specific application.
- mIoU: This is an indicator that indicates how much the predicted result overlaps with the ‘ground truth’. (Example: Calculate mIoU by evaluating how much the area where the model predicts a car overlaps with the actual car area.)
- Recall: Represents the proportion of data that the model predicts as a ‘ground truth’ among data that is actually a ‘ground truth’. (Example: The percentage of all cars in an image that the model classifies as a car.)
- Accuracy: Indicates how many predictions the model accurately made out of the total data. (Example: The percentage of all images correctly classified as cars or non-cars.)
- Precision: This refers to the ratio of cases where the model predicts a ‘ground truth’ to actually be the ‘ground truth’. (Example: The percentage of images predicted by the model to be cars that are actually car images.)
- mAP: This is an indicator that measures the model’s performance in terms of finding the ground truth. It represents the average value of AP, and the higher the value, the better the performance. (Example: This is calculated by taking into account the accuracy with which the model predicts for all cars and the proportion of actual cars.)
- F1-Score: represents the harmonic mean of precision and recall, showing how well the two metrics are balanced. (For example, in a project where both precision and recall are important, you can look at the F1-Score to assess the balance of the two metrics.)
Domain experts and citizen scientists can also intuitively interpret results through visual heatmaps
LISA calculates an anomaly score for each image or video frame. This score indicates how much the data in that area deviates from the normal range learned by the model, and when a high score is calculated, an alarm is sent to the user in real time.
Based on this, LISA creates a ‘heatmap’ that displays high-scoring areas in different colors. Higher scores are displayed in red, allowing users to distinguish them at a glance. By providing a visual heatmap like this instead of complex numbers or log data, it helps even domain experts or citizen scientists without AI knowledge easily interpret the results. For example, if a specific mechanical component failure or product defect is highlighted in red, the user can immediately inspect the spot and take action.
Heatmaps also show not only the specific location where anomalies are detected, but also how much the data deviates from normal, allowing users to easily determine the severity and priority of the problem.
Automatically generate evaluation and testing reports and dashboards
LISA automatically generates a detailed report after completing model validation and testing. It contains key information about the model, such as the algorithm used, learning preset settings, learning data composition, and confusion matrix. Through this, users can check the model’s precision and recall rate and establish strategies to improve the model’s weaknesses. Additionally, inference results for individual images are included, allowing users to further analyze issues occurring with specific images or classes.
Reports can be exported in HTML format. Anyone can easily view and share through a web browser. This helps clearly communicate project progress and promote collaboration among team members. HTML reports can be converted to other formats and used for various purposes.
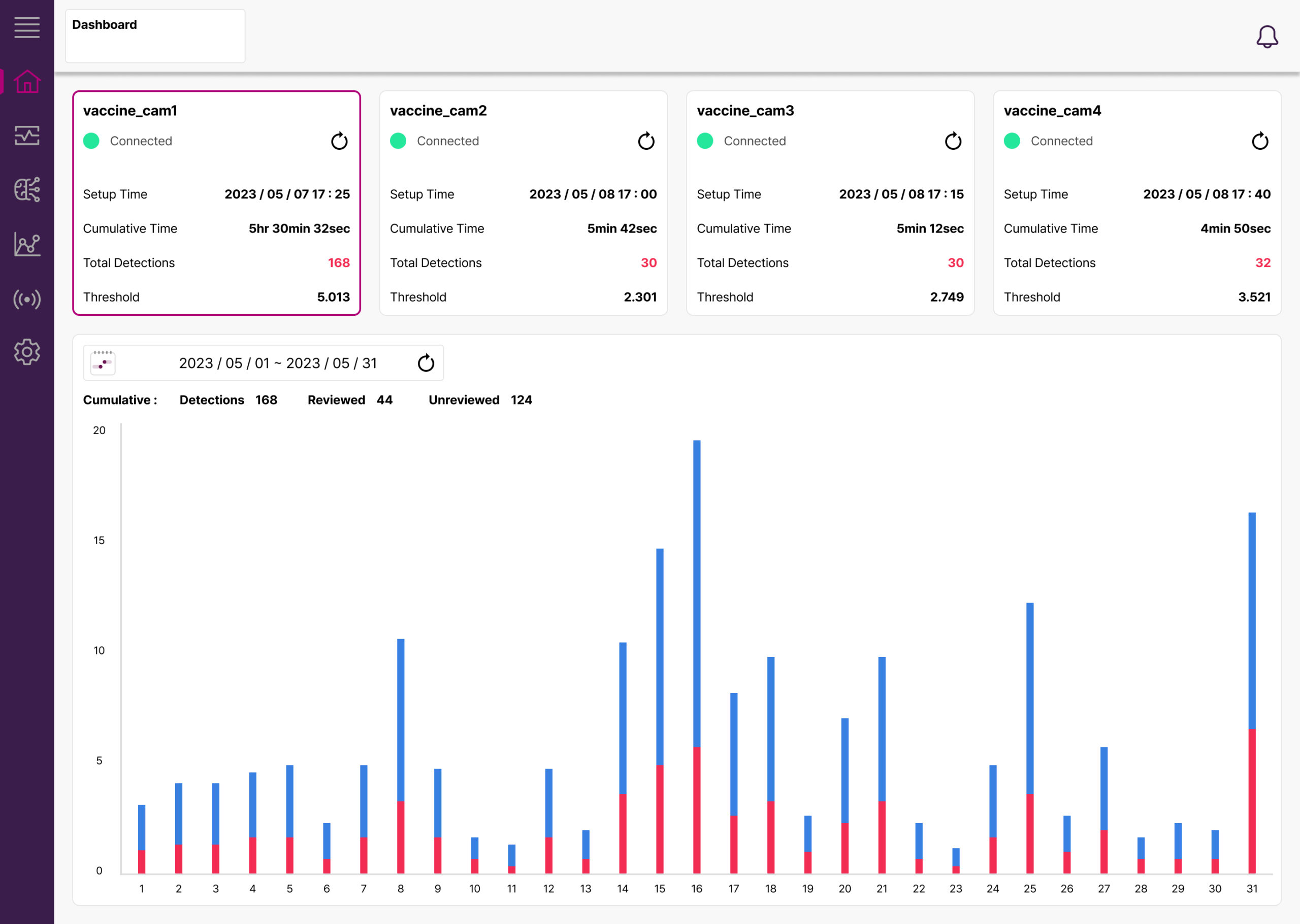
Keep up-to-date with graphs that update in real-time.
LISA provides real-time anomaly detection status visualized in graphs. It can be checked on a monthly/weekly/daily basis, allowing users to intuitively understand the total number of abnormalities detected and trends. This graph updates in real time as data is collected, so you always have the most up-to-date information. For example, you can easily identify a pattern of a sharp increase in the number of anomaly detections over a certain period of time, allowing you to quickly remediate or take preventative action.
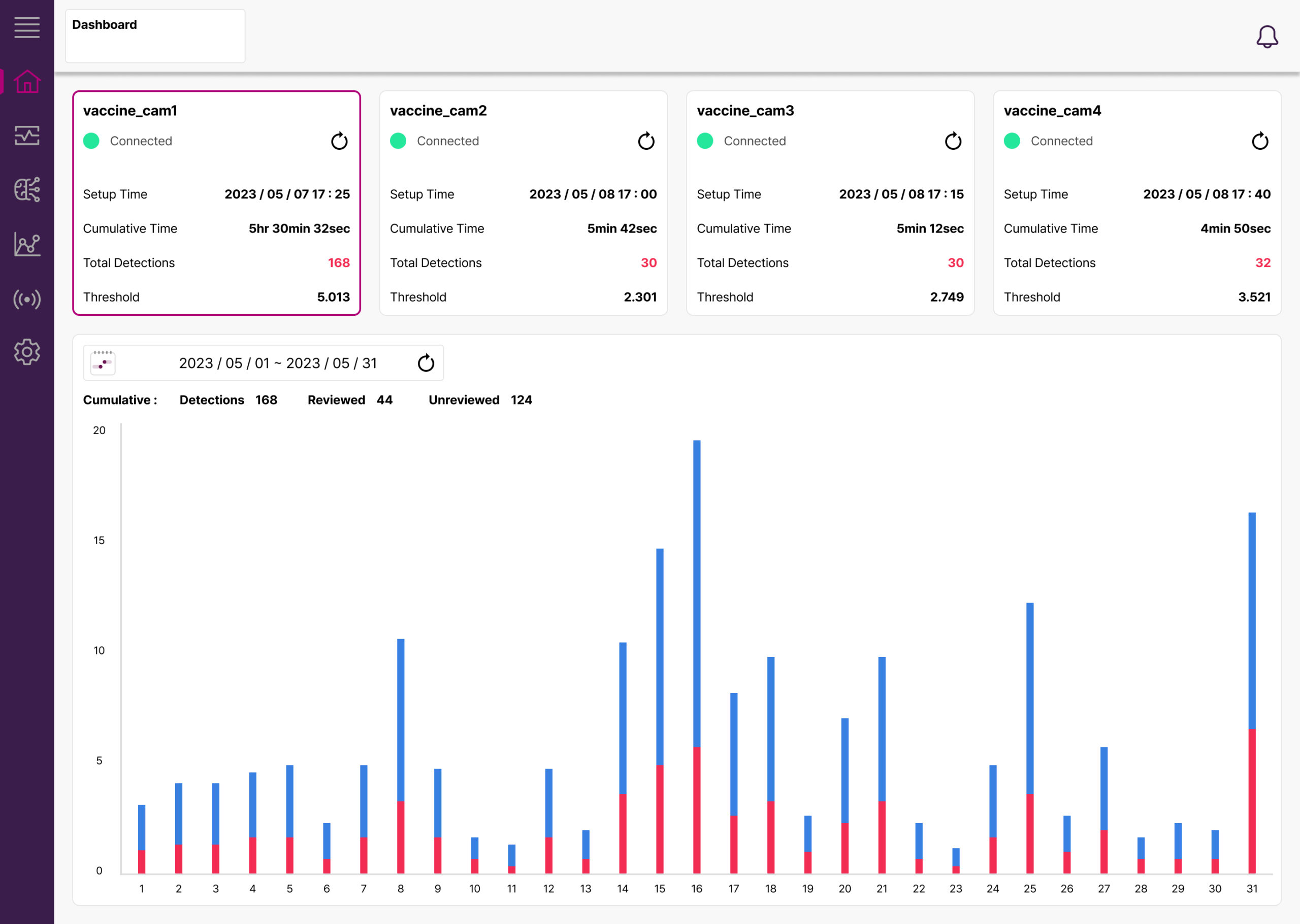
Achieve Your Business Goals
with a Convenient MLOps Platform
Achieve Your Business Goals with a Convenient MLOps Platform
A successful case of reducing ‘false-positives’ by applying a deep learning model to detect ‘type 1 errors’
Challenge If the test standard is set too sensitively to achieve 0%...
Success case of monitoring ‘data drift’ and performing predictive maintenance with a data quality index (DQI) model
Challenge Consistent optical images must be taken at all times to...
Configuring an AI model pipeline to detect robotic grasping anomalies in real time
Challenge Robot drops battery, causing downtime Difficulty utilizing...
Next Step →
With the help of LISA, gain a deep understanding of the performance of AI models and achieve your goals easily and quickly.