LISA
Unsupervised learning without labeling
Develop models quickly using only normal data using Anomaly Detector,
and ensure high usability in any process with free model pipeline configuration.
Develop models quickly using only normal data using Anomaly Detector, and ensure high usability in any process with free model pipeline configuration.
Develop models quickly with only normal data using Anomaly Detector
LISA features the Anomaly Detector algorithm, which utilizes label-free, unsupervised learning. The algorithm learns the underlying structure and patterns of the data to extract a feature map, which is then compared to the input data to calculate an outlier score. This effectively identifies outlier data that deviates from the normal range.
Users can cut the time and cost of acquiring and preprocessing anomalous data by half or more, as the Anomaly Detector develop models using only normal data. Deep learning can be implemented from the earliest stages of production, enabling rapid and efficient anomaly detection and quality control.
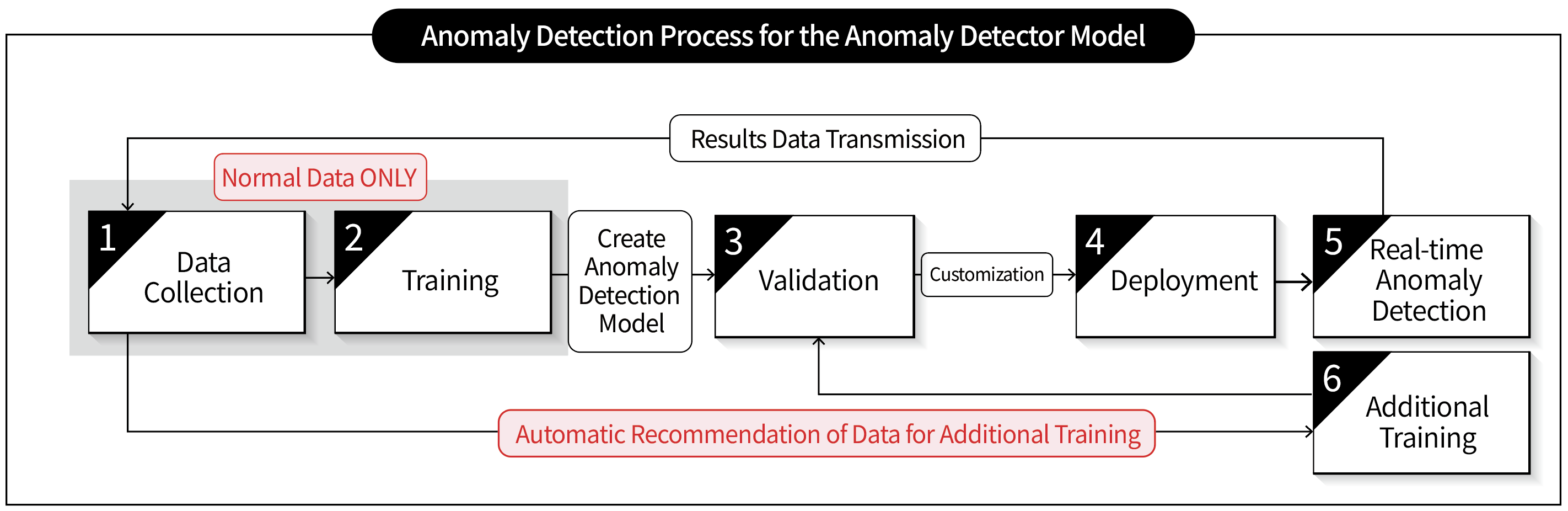
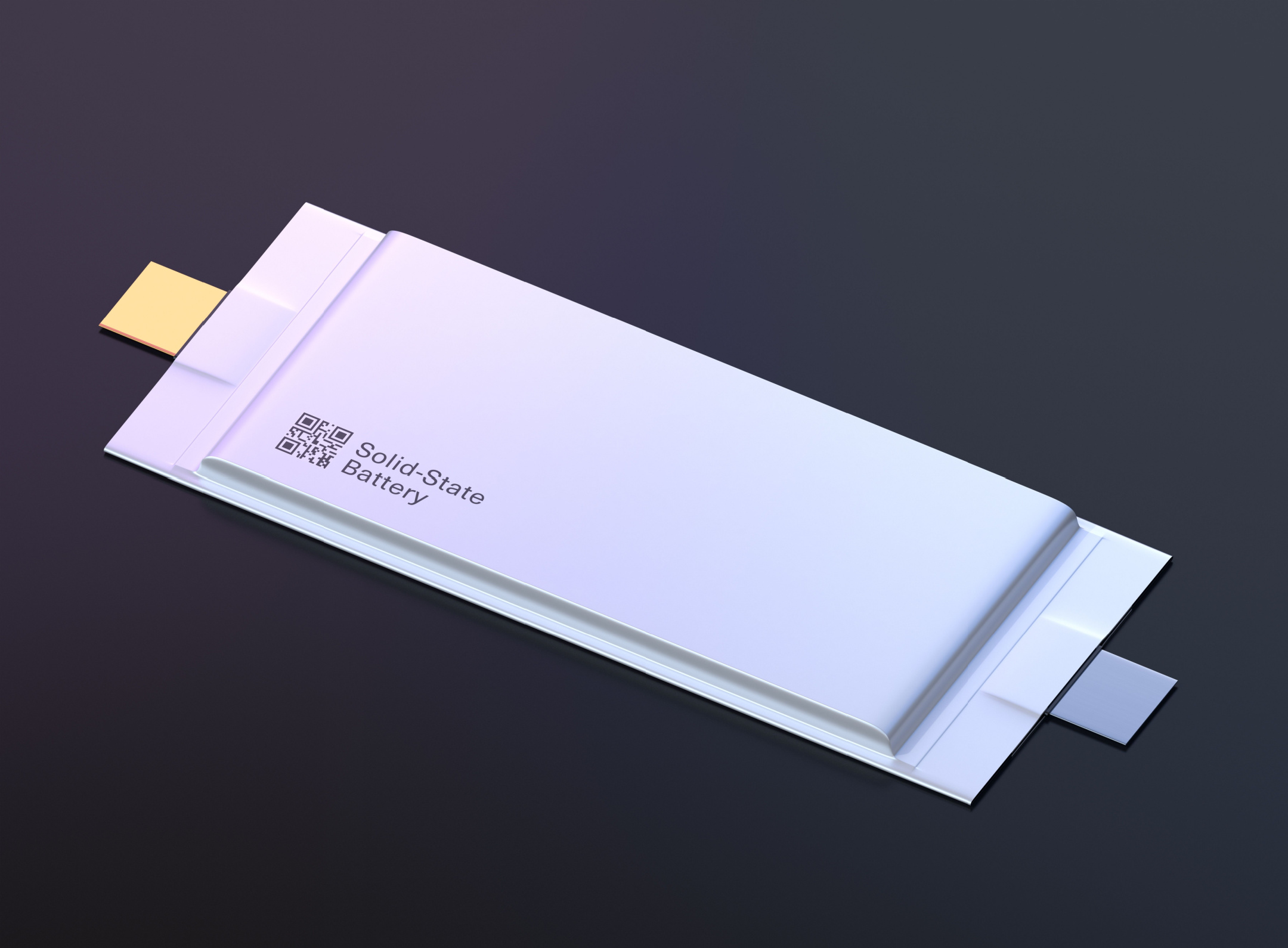
Anomaly Detector is particularly recommended for use in the following conditions.
✓ In cases where the product’s surface freely changes due to the use of thin or flexible materials
When a product has a complex geometry or varies from one product to another, it is difficult to cover all cases with traditional rule-based inspection methods. Anomaly Detector detects anomalies through differences from the learned normal pattern, so it can be used effectively even for products with high geometric complexity.
✓ When it’s difficult to establish criteria for traditional rule-based inspections
In the absence of a normalized baseline, Anomaly Detector learns the normal range of your data on its own and determines anomalies based on that. This greatly reduces the time and effort required to set up unclear rules.
✓ When it is difficult to predict new types of defects
Proactively predicting new types of defects that may arise from market changes or product upgrades is very challenging. Anomaly Detector can detect new forms of defects as outliers, even if they are not in the training data, allowing you to react quickly to changes.
✓ New production and class imbalance issues
In the early days of new production, you often don’t have enough abnormal data. Anomaly Detector can be trained with only normal data and can be applied effectively in the early stages of new production. After production has been running for a while and a large amount of abnormal data has been accumulated, you can use a supervised learning model, which has a much higher accuracy.
Speed up training and inspection with ROI specification
Before training on normal data, you can easily designate areas across an image or video where anomalies are more likely to be detected using just your mouse in a user-friendly UI. This helps improve anomaly detection accuracy. Use the polygon tool to accurately select regions of interest (ROIs) with complex and irregular shapes, and easily remove unwanted background or noise. This reduces the amount of data that needs to be processed during the training process, speeding up training and inspection time.
Build your ML pipeline with five built-in models
Several types of models are built-in, including Object Detector, Segmentation, Classification, and OCR. If you already have abnormal data, or if the quality control rules applied in your existing production process are relatively clear, you can immediately run the built-in models provided by LISA to quickly analyze your data.
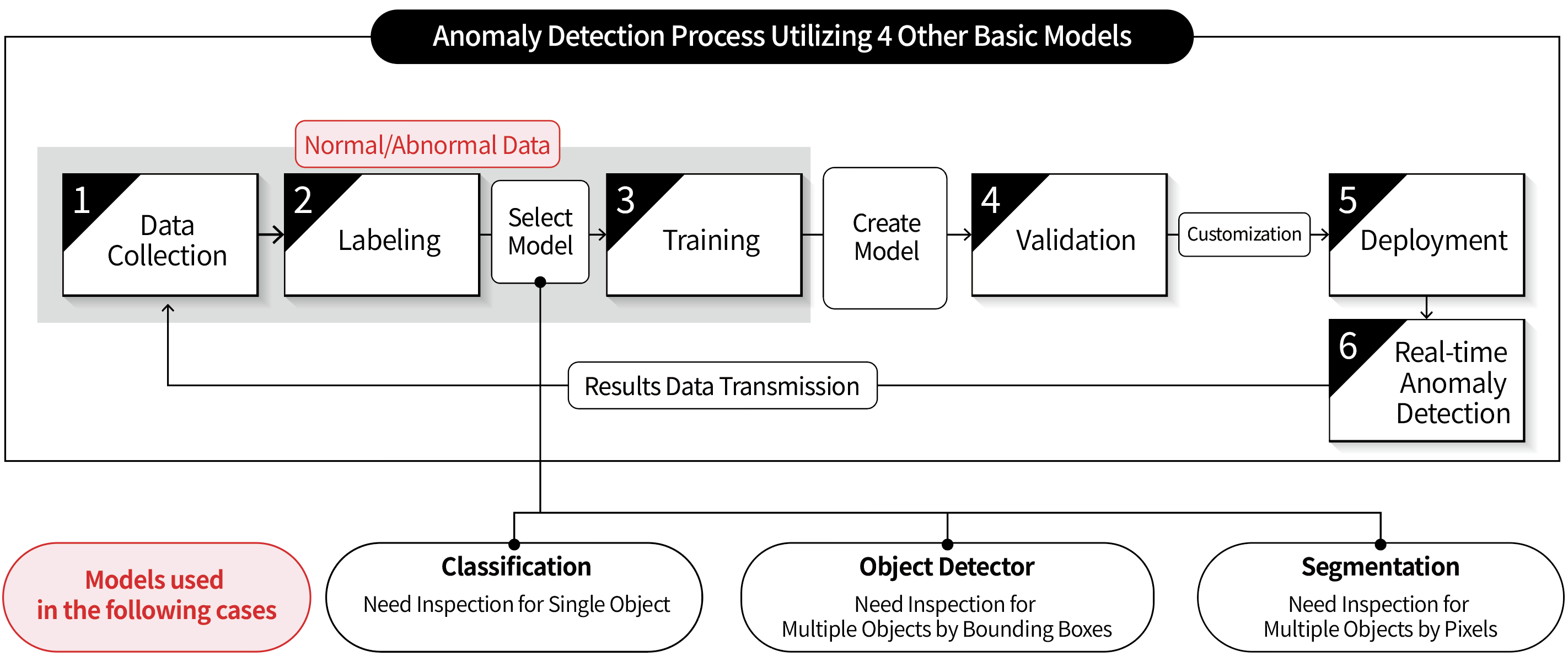
You can also combine two or more models to form a pipeline. For example, you can arrange to first identify surface defects with the Anomaly Detector and then further classify the type of defect with the Classificaion model, or to first identify objects that need to be quality judged with the Object Detector and then determine outliers with the Anomaly Detector. By combining the strengths of different models, especially in complex processes, you can achieve more precise judgment results.
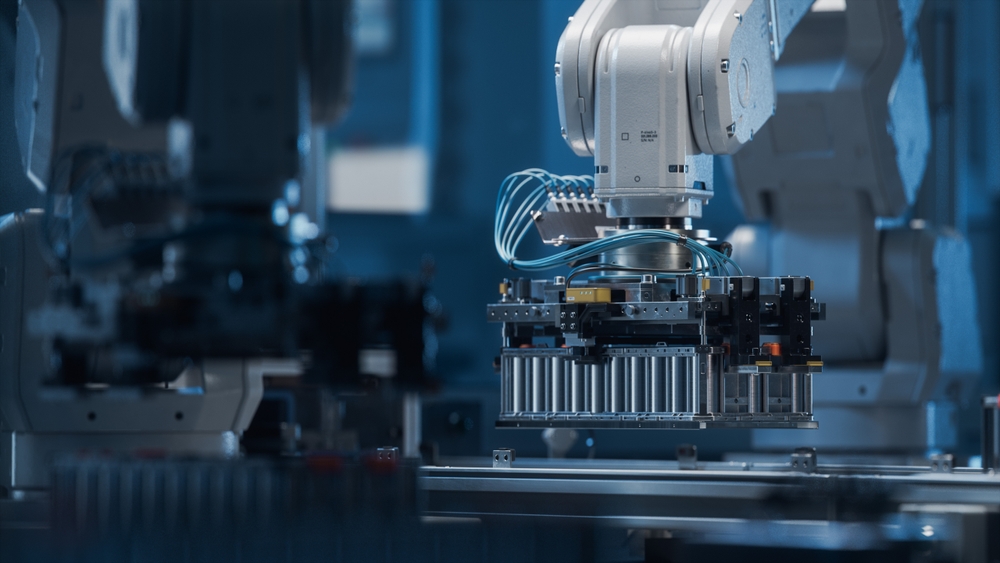
Make supervised learning easy with an intuitive toolbar
When you set up a pipeline with models that require supervised learning, you can easily label them with an intuitive toolbar.
- Select filters and classes
◦ Predefine different object types
◦ Select and view specific images - Shape drawing tools
◦ Square, rectangle, circle, ellipse, polygon, etc. - Brush tools
◦ Easy and quick labeling - Adjust object transparency
◦ Visually clarify where images are overlapped - Undo and redo
◦ Fix labeling mistakes - Page flipping
◦ Quickly review and edit multiple images
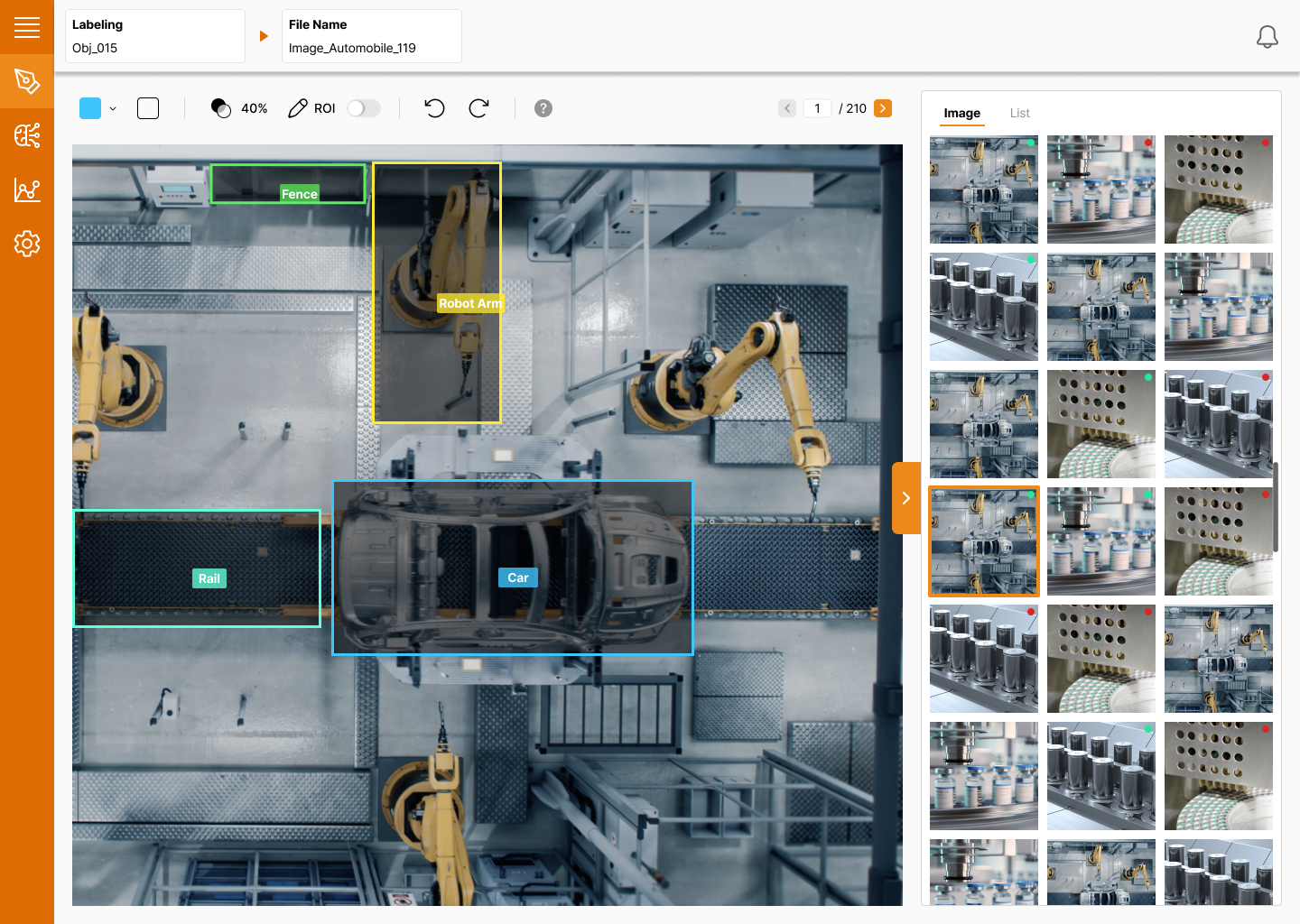
See your labeling status at a glance with the sliding drawer
LISA provides a sliding drawer to effectively categorize and manage your labeled images. It’s easy to find the images you need and know at a glance whether they’re labeled and in what class, which is invaluable when working with large datasets. Take the pain out of data preparation with LISA.
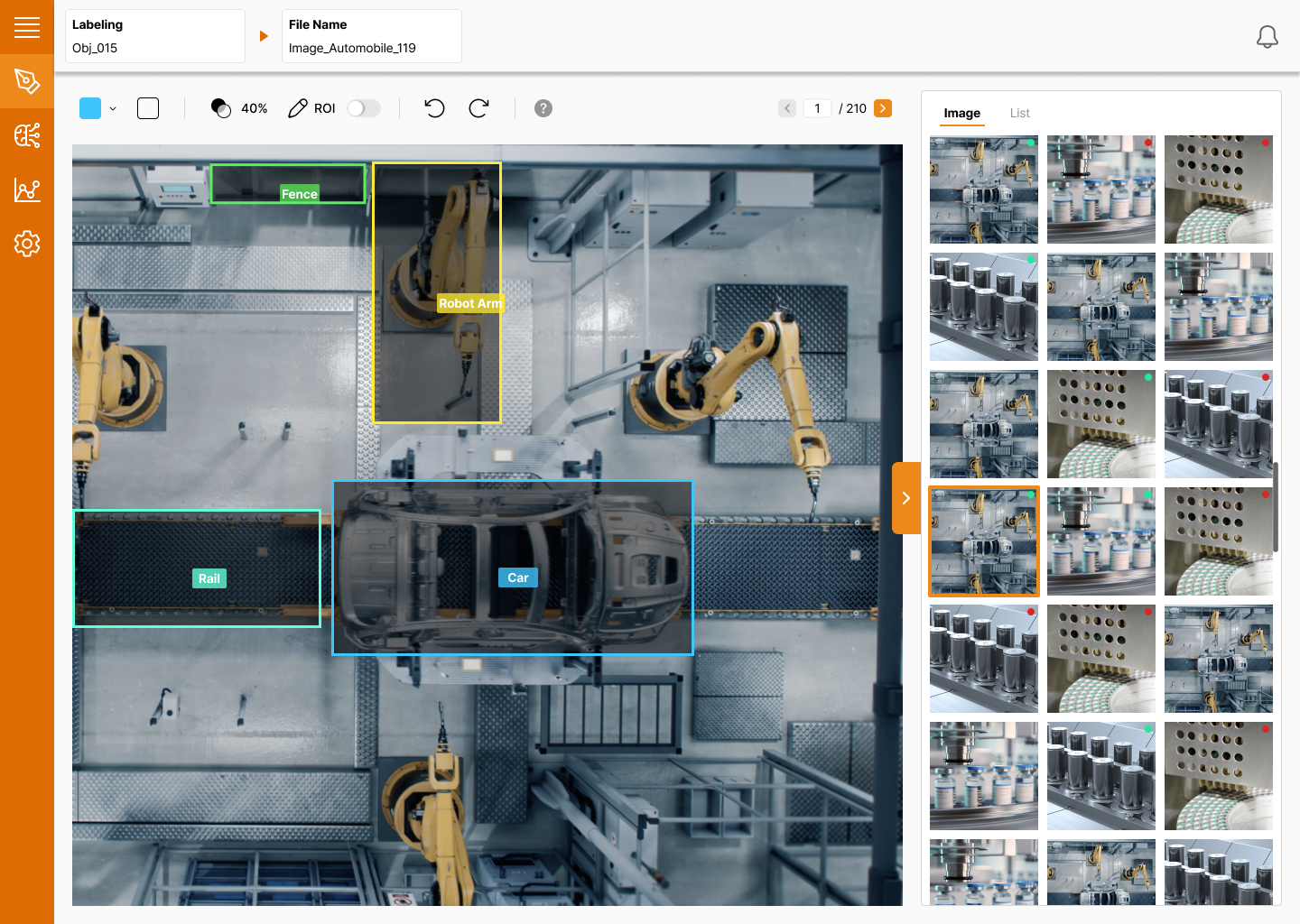
Achieve Your Business Goals
with a Convenient MLOps Platform
Achieve Your Business Goals with a Convenient MLOps Platform
AI Inspection with 86% Cost Reduction Using On-Device AI
Challenge Defects caused by external contaminants are unpredictable in shape,...
Transforming Battery Manufacturing with AI Inspection & Data Automation
From Manual Inspections to Smart Factories: The Evolution of Battery...
A 3-Step AI Inspection Pipeline for Rubber Sheet Defects: Automating Detection, Classification, and Location Analysis
Challenge In the tire manufacturing process, rubber sheets produced during...
Next Step →
Leverage LISA’s sophisticated AI pipeline to maximize quality control and increase production efficiency.