A 3-Step AI Inspection Pipeline for Rubber Sheet Defects: Automating Detection, Classification, and Location Analysis
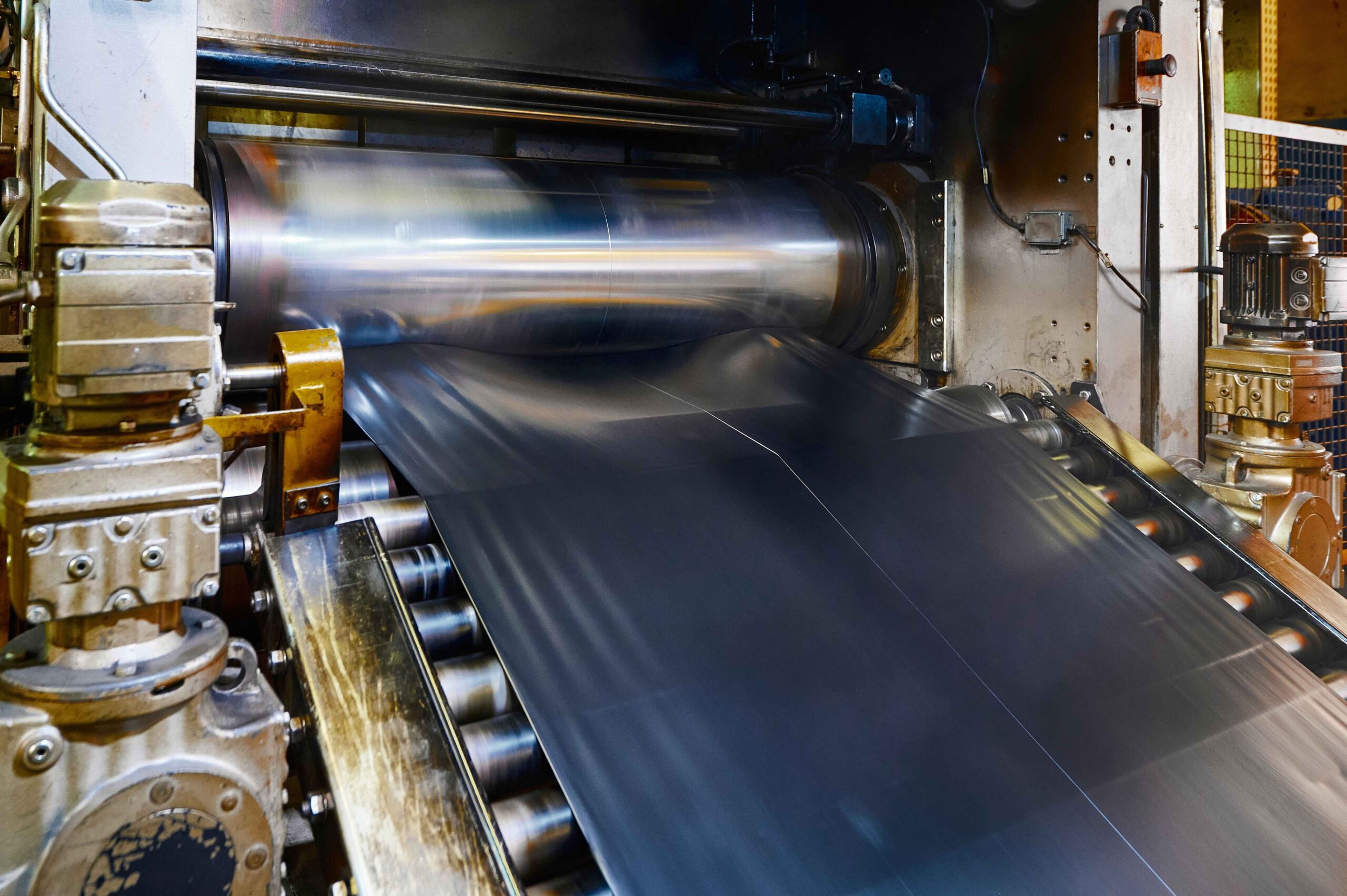
Challenge
In the tire manufacturing process, rubber sheets produced during the calendering process lacked an automated quality inspection system and relied heavily on manual visual inspections.
This resulted in three significant issues:
- Manual vision inspection was prone to human error leaving some defects undetected.
- Substantial waste of finished products caused by undetected defects in the rubber sheets.
- There was no systematic method to analyze the types or location of defects over time to find causes of defects.
Approach
AHHA Labs developed a streamlined 3-step quality inspection pipeline to address these challenges:
- Anomaly Detection
Real-time identification of defective rubber sheets. - Classification
Automated categorization of defect types (e.g., contamination, scratches, or holes). - Defect Location Calculation
Leveraging PLC data to determine the exact location of defects.
The inspection results were used to control the PLC system, ensuring rapid adjustments to the process. A comprehensive monitoring dashboard was also developed to visualize inspection results and access detailed defect histories.
Result
- 75% Faster AI Deployment
Leveraging anomaly detection models trained on normal data, AHHA Labs reduced deployment time by 75% compared to competitors. - 99.9% Accuracy
The system achieved near-perfect accuracy in detecting and classifying even complex, irregular defect patterns. - Improved Efficiency
Automated PLC control based on inspection results significantly enhanced production speed. - Seamless Integration
Full compatibility with the existing MES system ensured smooth operations and real-time monitoring. - Defect Insights
Analysis of defect types provided valuable insights to optimize pre-calendering processes.
Full Story
Every tire begins its journey as a mixture of rubber compounds. These are carefully mixed, coated over thin mesh layers, and rolled through a series of machines to produce smooth rubber sheets. These sheets are later cut, shaped into rolls, inflated, and molded into the final tire shape. This process is referred to as calendering.
Global Tire Company A has built its global reputation with superb product qualities and it held itself to extreme strict quality standards. However, the calendering process presented unique challenges in quality inspection. The defects were visually inspected by skilled workers between the rolling and cutting stages.
These workers were highly experienced veterans claiming relatively high wages, yet they had to withstand the harsh environment for 8 hours straight. Their efficiency would be impacted by fatigue. Furthermore, the client was wary that the tribal knowledge retained in these workers individually was hard to be transferred to or replicated by the next generation of workers.
Meanwhile, introducing AI inspection appeared to be impractical given that there was barely any space to place cameras or lights.
To improve inspection efficiency and accuracy, the company collaborated with AHHA Labs to automate the process using AI. The goal was to detect defects in the rubber sheets, pinpoint their locations, and classify their types automatically. This would allow downstream operators to remove defective areas and continue producing defect-free rubber sheets for tire manufacturing.
The 3-Step Inspection Pipeline with LISA + Data CAMP
AHHA Labs began by installing cameras and lighting equipment on either side of the rubber sheets. The captured images, along with machine data from the PLC system, were transmitted to LISA, AHHA Labs’ industrial AI platform, and the Data CAMP, an industrial big data platform.
The data was processed through a 3-step pipeline:
- Anomaly Detection
Identifies defects using a model trained exclusively on normal data. - Classification
Categorizies defect types, such as contamination, scratches, or holes, using a classification model. - Defect Localization
Determins the precise defect location based on PLC machine data.
The processed data was sent back to the PLC system, which executed necessary control commands to adjust the production process. AHHA Labs also proided a monitoring dashboard, enabling operators to view detailed inspection results in real time. The dashboard supported remote monitoring via external networks, further enhancing its utility.
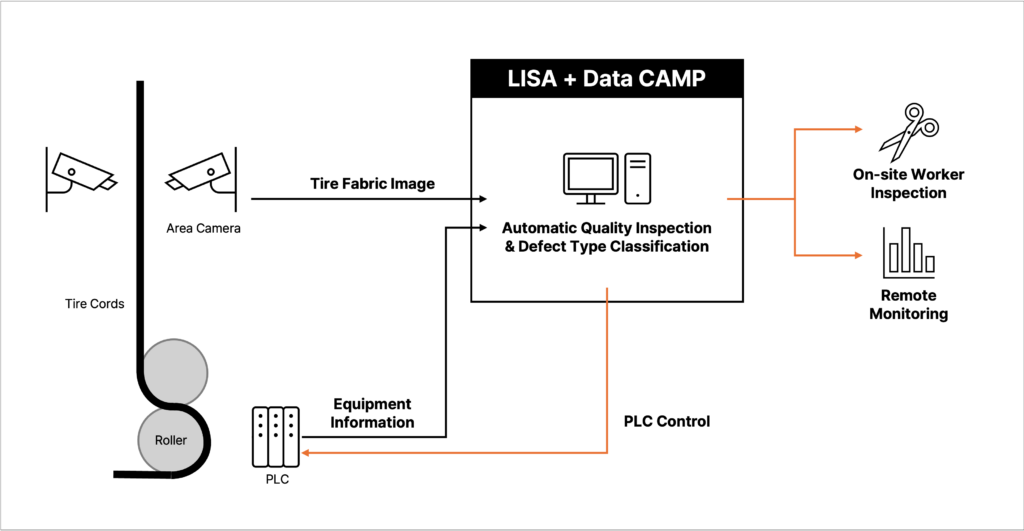
LISA and Data CAMP seamlessly collaborate to detect defects, pinpoint their locations, classify types, and enable real-time monitoring. Image Credit: AHHA Labs
From Manual Visual Inspection to AI-Powered Automation
The pilot system achieved an impressive 99.9% accuracy in detecting and classifying defects, practically eliminating reliance on manual visual inspections. This improvement led to:
- Lower inspection costs.
- Higher production efficiency.
- Valuable insights into the root causes of defects, enabling further process optimization.
Expansion to Mass Production
As of October 2024, AHHA Labs and Company A are evaluating the expansion of this system to production lines across Company A’s global factories.
🚀 Why Work with AHHA Labs?
Demonstrated expertise with a 65% conversion rate from pilot to mass production, significantly surpassing industry peers.
(According to the 2023 BCG report, only 16% of companies have achieved their AI-related goals.)
Industry success stories, including:
- Predictive Maintenance: Early detection of equipment anomalies using vibration sensor data.
- Robotics Pipeline: Successful anomaly detection in robotic gripper operations through AI model pipelines.
Collaborate with AHHA Labs to transform your manufacturing processes with cutting-edge AI solutions!
Boosting Control Efficiency of Battery Assembly Lines by 300% Through a Remote Monitoring System
Challenge The assembly lines for EV batteries are massive and highly complex, requiring multiple personnel to monitor equipment status and respond to alarms....
Detecting Inspection Data Drift Caused by Material Changes: A Case Study on the Data Quality Index (DQI) Model
Challenge In manufacturing, consistently capturing high-quality optical images is essential for training machine learning models and ensuring high...
Predicting Motor Failures with Vibration Sensor Data (Predictive Maintenance)
Challenge Previously, vibration sensors were installed on power plant motors, and inspections were conducted based on predefined rules. However, since...
A successful case of reducing ‘false-positives’ by applying a deep learning model to detect ‘type 1 errors’
Challenge If the test standard is set too sensitively to achieve 0% ‘false-negative’, ‘false-positive (type 1 error)’ increases. Inefficiency due to...
Success case of monitoring ‘data drift’ and performing predictive maintenance with a data quality index (DQI) model
Challenge Consistent optical images must be taken at all times to properly train the model and increase the accuracy of quality control However, if...
Configuring an AI model pipeline to detect robotic grasping anomalies in real time
Challenge Robot drops battery, causing downtime Difficulty utilizing existing machine learning solutions due to 'class imbalance' problem Difficulty...
How the Anomaly Detector model was used to automate the quality control process using only normal data
Challenge Pouch-type batteries have flexible surface geometry, complicating defect identification. This makes it challenging to use existing...
Implementing Quality Control in Multi-Product Small-Batch Production through the Utilization of Data CAMP’s ‘RECIPE’ feature
1. Challenge Managing Varied Quality Standards for Each Model without a Centralized History View The latest trend in manufacturing revolves around the...
Data Integration: Managing Inspection Data by Barcode Number for a Secondary Battery Manufacturer with Data CAMP
1. Challenge Lack of a production history management solution to manage product inspection data by barcode number In 2015, the introduction of the 21700...