Boosting Control Efficiency of Battery Assembly Lines by 300% Through a Remote Monitoring System
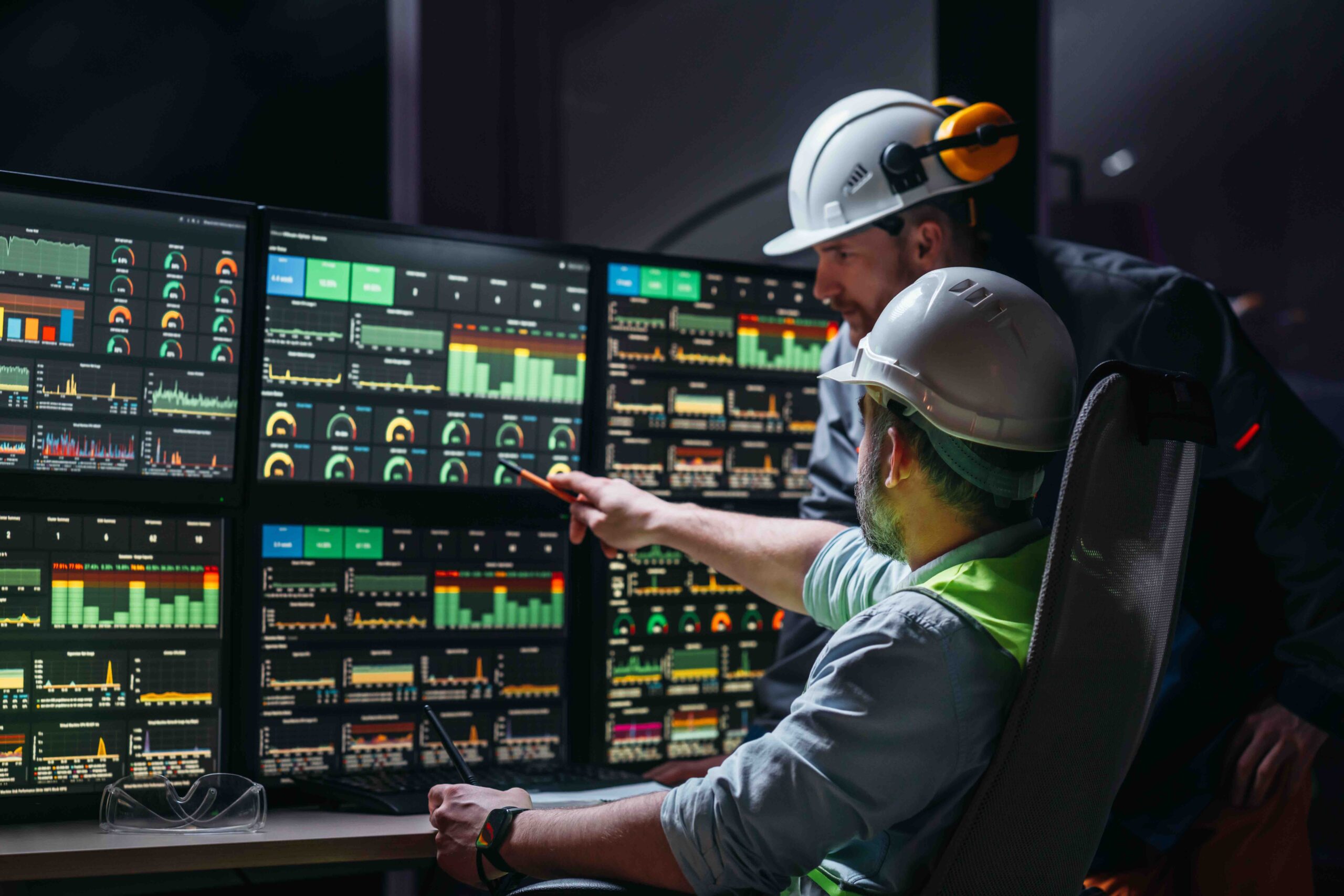
Challenge
- The assembly lines for EV batteries are massive and highly complex, requiring multiple personnel to monitor equipment status and respond to alarms.
- However, a predominantly large portion of the alarms are minor incidents that can be resolved with simple resets without the need for physical intervention.
- Goal: Streamline operations by implementing a remote monitoring and control system to reduce unnecessary labor and improve efficiency.
Approach
- Data Consolidation through Data CAMP
Centralized the data collected from various equipment using Data CAMP, AHHA Labs’ big data solution for manufacturers, to transmit large volumes of data effeciently to a central control tower. - Dashboard for Comprehensive Monitoring
Built an intuitive dashboard that enables clients to monitor the equipment status and receive real-time alerts. - Remote PLC Control: When an anomaly is detected, Data CAMP takes over PLC control authority, enabling customers to remotely operate equipment directly from the central control tower.
Result
- Implementation Time: 1.5 months
- Additional Hardware: One switch hub for connection
- Outcome: Reduced monitoring staff by 67%
- Future Goal: Fully automate alarm responses through AI pattern analysis
Full Story
Eliminating Inefficiencies with a Remote Monitoring System
The assembly lines for electric vehicle batteries are enormous, with each piece of equipment spanning tens of meters. These large setups are often interconnected, creating a highly complex system.
Given the high-speed, high-precision nature of battery assembly, frequent warning alarms are inevitable. Each time an alarm goes off, personnel must physically inspect the equipment, identify the issue, and resolve it.
Due to the scale and complexity of the equipment, a significant workforce is required to continuously monitor and manage operations.
However, a substantial portion of these alarms can be resolved with simple actions, such as equipment resets. The inefficiency of physically traveling between equipment to address such minor issues was a major bottleneck.
Company E, which produces secondary batteries for electric vehicles, sought to eliminate this inefficiency by implementing a remote monitoring and control system for their battery assembly equipment.
Centralized Data Collection and Remote PLC Control
The AHHA Labs team developed a system designed to provide centralized monitoring and remote PLC control of all equipment.
- Data Collection
Data CAMP, AHHA Labs’ industrial big data solution, was installed on the PCs of each piece of equipment. This solution collected equipment data and transmitted it to a central monitoring PC. - Dashboard Visualization
The collected data was displayed on an integrated dashboard. The main screen allowed real-time monitoring of the operational status and alarm information in one glance. To optimize network traffic for efficiency, detailed information was provided when the user selected specific equipment. - Remote PLC Control
In cases of anomaly detection, the system enabled remote PLC control. To prevent control conflicts among the complex machinery, AHHA Labs carefully designed and tested operational scenarios before implementing the governance system onto the PLC.
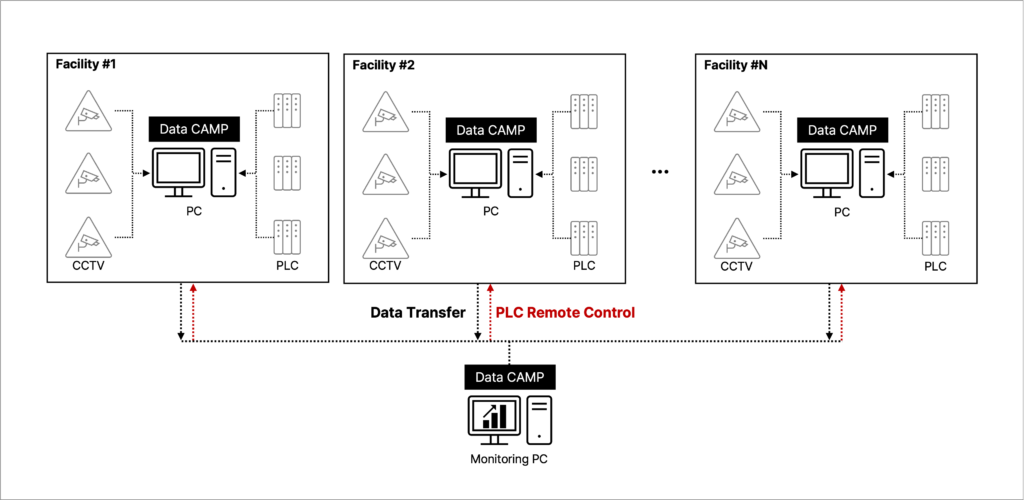
Data CAMP, AHHA Labs’ industrial big data solution, collects equipment data, transmits it to a central monitoring PC, and performs remote monitoring and PLC control while maintaining the existing infrastructure. The web-based Data CAMP solution enabled the system to be implemented in just 1.5 months. Image credit: AHHA Labs
Achieved Implementation in Just 1.5 Months, Reducing Labor Costs by 67%
While similar control automation programs typically require most solution providers six months or more to develop, the web-based Data CAMP solution was up and running in within just 1.5 months.
Additionally, leveraging a data-centric solution eliminated the need for additional software development. The system maintained the existing network infrastructure, requiring only a single switch hub as new hardware—dramatically reducing hardware investment costs.
The system successfully implemented all the target functionalities, including data collection, monitoring, detailed equipment information retrieval, and remote PLC control. This optimization reduced monitoring staff requirements by 67%.
Future Goals: Fully autonomous monitoring system with AI
In the future, the company plans to analyze alarm patterns using AI and fully automate alarm handling, achieving a fully autonomous monitoring system.
About Data CAMP
Features:
- Supports various industrial communication protocols, enabling unified data collection, transmission, and storage across heterogeneous equipment.
- Enables warning alarm notifications and remote equipment control via PLC communication.
- A data-driven solution allows for flexible addition and removal of equipment, data, and functionalities without additional software development.
- Recipe-based no-code block-coding UI makes it easy to build data pipelines optimized for manufacturing processes.
- Customizable dashboards and Statistical Process Control (SPC) capabilities for pattern analysis and anomaly detection in real time.
Data CAMP seamlessly collects all manufacturing data, integrates with existing systems, and automates analysis for enhanced operational efficiency.
Detecting Inspection Data Drift Caused by Material Changes: A Case Study on the Data Quality Index (DQI) Model
Challenge In manufacturing, consistently capturing high-quality optical images is essential for training machine learning models and ensuring high...
Predicting Motor Failures with Vibration Sensor Data (Predictive Maintenance)
Challenge Previously, vibration sensors were installed on power plant motors, and inspections were conducted based on predefined rules. However, since...
A successful case of reducing ‘false-positives’ by applying a deep learning model to detect ‘type 1 errors’
Challenge If the test standard is set too sensitively to achieve 0% ‘false-negative’, ‘false-positive (type 1 error)’ increases. Inefficiency due to...
Success case of monitoring ‘data drift’ and performing predictive maintenance with a data quality index (DQI) model
Challenge Consistent optical images must be taken at all times to properly train the model and increase the accuracy of quality control However, if...
Configuring an AI model pipeline to detect robotic grasping anomalies in real time
Challenge Robot drops battery, causing downtime Difficulty utilizing existing machine learning solutions due to 'class imbalance' problem Difficulty...
How the Anomaly Detector model was used to automate the quality control process using only normal data
Challenge Pouch-type batteries have flexible surface geometry, complicating defect identification. This makes it challenging to use existing...
Implementing Quality Control in Multi-Product Small-Batch Production through the Utilization of Data CAMP’s ‘RECIPE’ feature
1. Challenge Managing Varied Quality Standards for Each Model without a Centralized History View The latest trend in manufacturing revolves around the...
Data Integration: Managing Inspection Data by Barcode Number for a Secondary Battery Manufacturer with Data CAMP
1. Challenge Lack of a production history management solution to manage product inspection data by barcode number In 2015, the introduction of the 21700...