Detecting Inspection Data Drift Caused by Material Changes: A Case Study on the Data Quality Index (DQI) Model
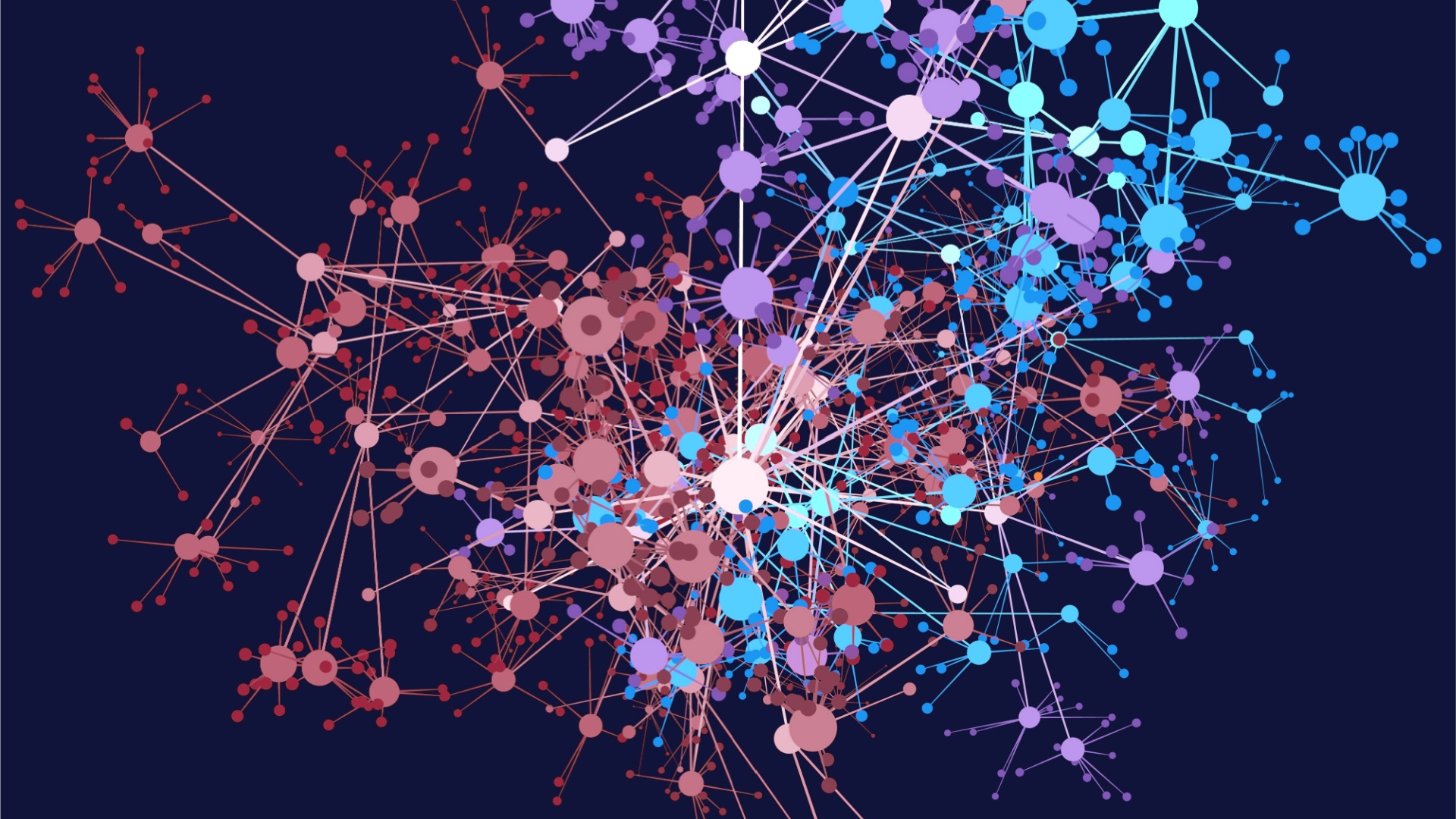
Challenge
- In manufacturing, consistently capturing high-quality optical images is essential for training machine learning models and ensuring high accuracy in defect classification. However, fluctuations in production environments often lead to “data drift,” resulting in frequent false positives (NG classifications), even when no actual defects are present. When percentage of false positives is high, manufacturers often end up relying on a large team of experienced QA experts to review all the rejected items to minimize scrap.
- A cost-effective and simple solution was required to monitor the quality of inspection images in real time, ensuring their alignment with training data standards.
Approach
- To tackle this issue, AHHA Labs implemented the Data Quality Index (DQI), a deep learning model that quantifies deviations between newly captured inspection images and the reference images used during model training.
- Additionally, the Data CAMP dashboard was upgraded with customizable DQI monitoring features, tailored to accommodate the unique conditions of each manufacturing environment. For example,
Result
- When production materials were changed, differences in surface reflectivity and contour shapes caused outliers to appear on the DQI section of the dashboard. This early detection alerted the team to deviations from the normal data distribution range.
- By promptly reviewing the flagged images, the team identified potential adjustments to the optical setup and took immediate action, ensuring seamless quality inspections and maintaining production yield without downtime.
Full Story
The Data Quality Index (DQI) is AHHA Labs’ proprietary deep learning model designed to monitor the quality of input data. If newly captured images significantly deviate in focus or brightness from reference images—making accurate defect classification impossible—the model triggers an alert for the user.
Users can review flagged outliers (red dots outside the ellipse on the dashboard) by simply clicking on them. If an image is deemed acceptable, the user can click the “Classify as Normal” button. This action recalibrates the upper and lower boundaries of the normal range and dynamically adjusts the ellipse displayed on the dashboard. In essence, the dashboard allows intuitive outlier labeling and centralized management.
📌 Curious to learn more about DQI? Explore the detailed article below!
For instance, a manufacturer of EV batteries noticed red outlier dots appearing outside the normal range on their DQI dashboard one day.
The AHHA Labs team immediately investigated the root cause and discovered that changes in production materials had altered surface reflectivity and contour shapes, impacting the quality of captured images.
If these data changes had gone unnoticed, the quality inspection process could have been compromised over an extended period, potentially affecting production yield.
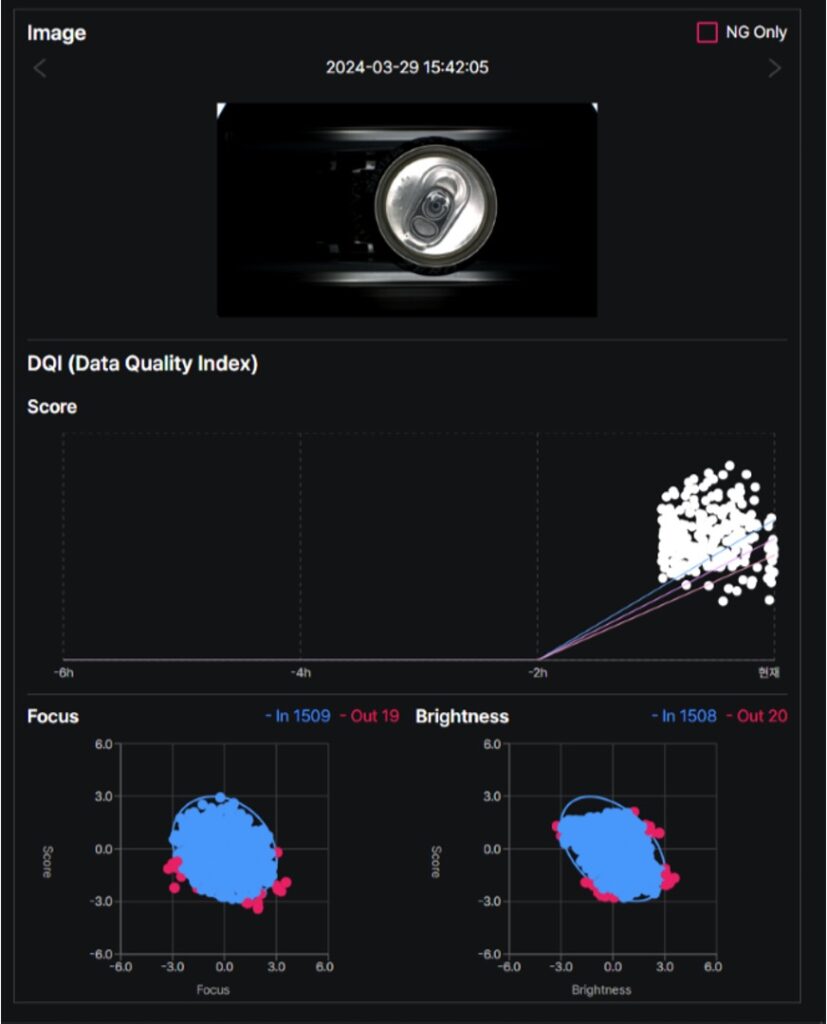
The DQI dashboard example shows the relationship between focus or brightness (x-axis) and DQI scores (y-axis). Blue dots inside the ellipse represent normal data, while red dots outside indicate outliers. By analyzing this visualization, users can identify why the model classified certain images as abnormal. The dashboard also simplifies outlier management with just a few clicks. Image Credit: AHHA Labs
By monitoring DQI, teams can detect and resolve data drift issues early. For example, as described above, on-site personnel can promptly review flagged images and determine whether adjustments to the optical setup, such as lighting or camera settings, are necessary to maintain inspection accuracy.
Predicting Motor Failures with Vibration Sensor Data (Predictive Maintenance)
Challenge Previously, vibration sensors were installed on power plant...
A successful case of reducing ‘false-positives’ by applying a deep learning model to detect ‘type 1 errors’
Challenge If the test standard is set too sensitively to achieve 0%...
Success case of monitoring ‘data drift’ and performing predictive maintenance with a data quality index (DQI) model
Challenge Consistent optical images must be taken at all times to...
Configuring an AI model pipeline to detect robotic grasping anomalies in real time
Challenge Robot drops battery, causing downtime Difficulty utilizing...
How the Anomaly Detector model was used to automate the quality control process using only normal data
Challenge Pouch-type batteries have flexible surface geometry,...