LISA
Automated & Customized
Quickly develop models with custom presets tuned by AI experts,
and flexibly enhance model performance by adjusting thresholds according to changing field conditions.
Quickly develop models with custom presets tuned by AI experts, and flexibly enhance model performance by adjusting thresholds according to changing field conditions.
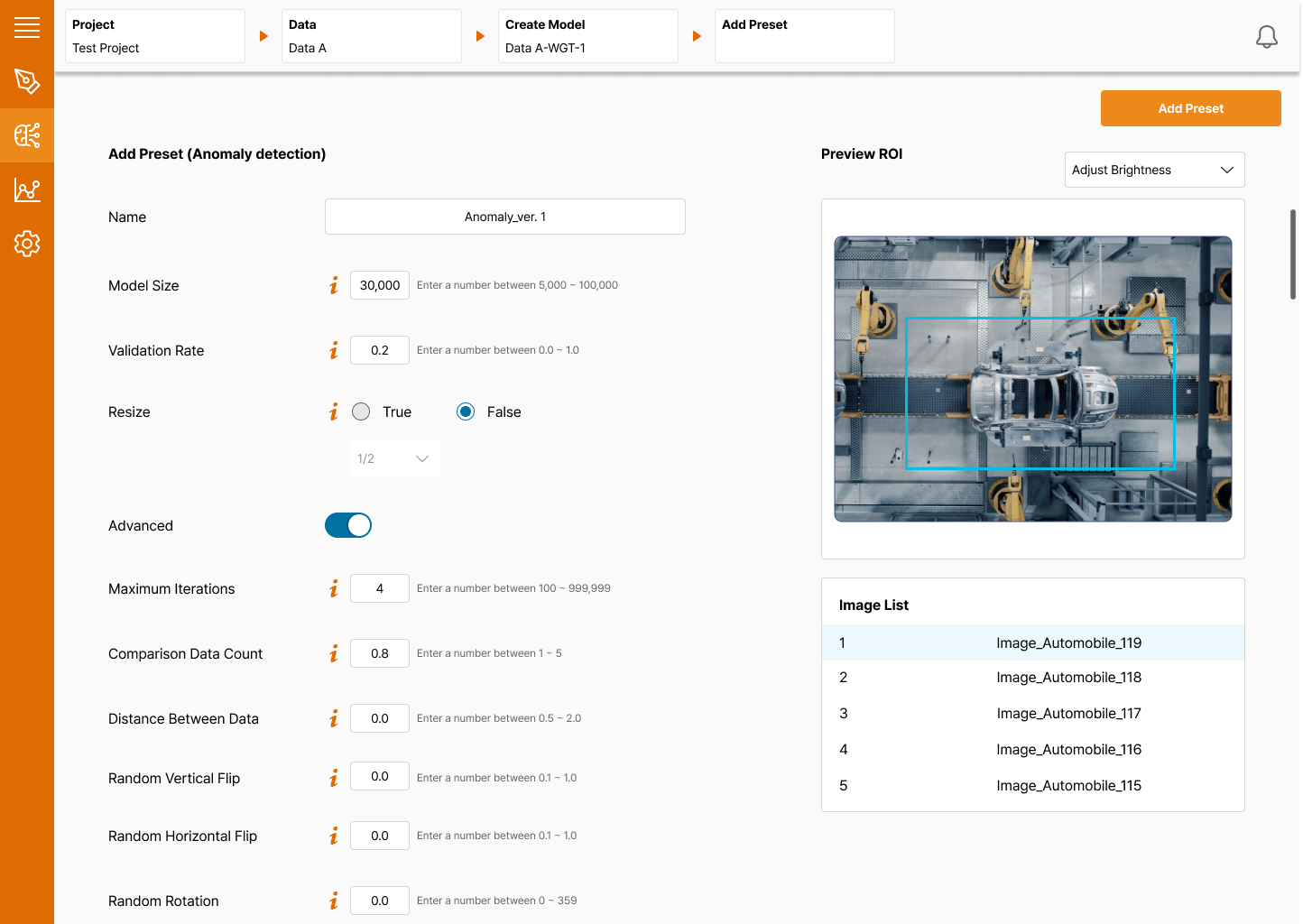
Custom learning presets pre-tuned by machine learning engineers
LISA provides a wide range of learning preset options, including model size, number of learning repetitions, verification cycle, high-precision option, weight update rate, weight reduction rate, learning method (context/texture priority), evaluation data rate, image scale, vertical/horizontal flip, rotation range, brightness control, and more. By using recommended presets derived from extensive prior experiments by machine learning engineers as the default, LISA helps users create optimal models even without understanding the exact impact of each element. If more detailed adjustments are needed, each learning preset can be easily modified through the user-friendly UI.
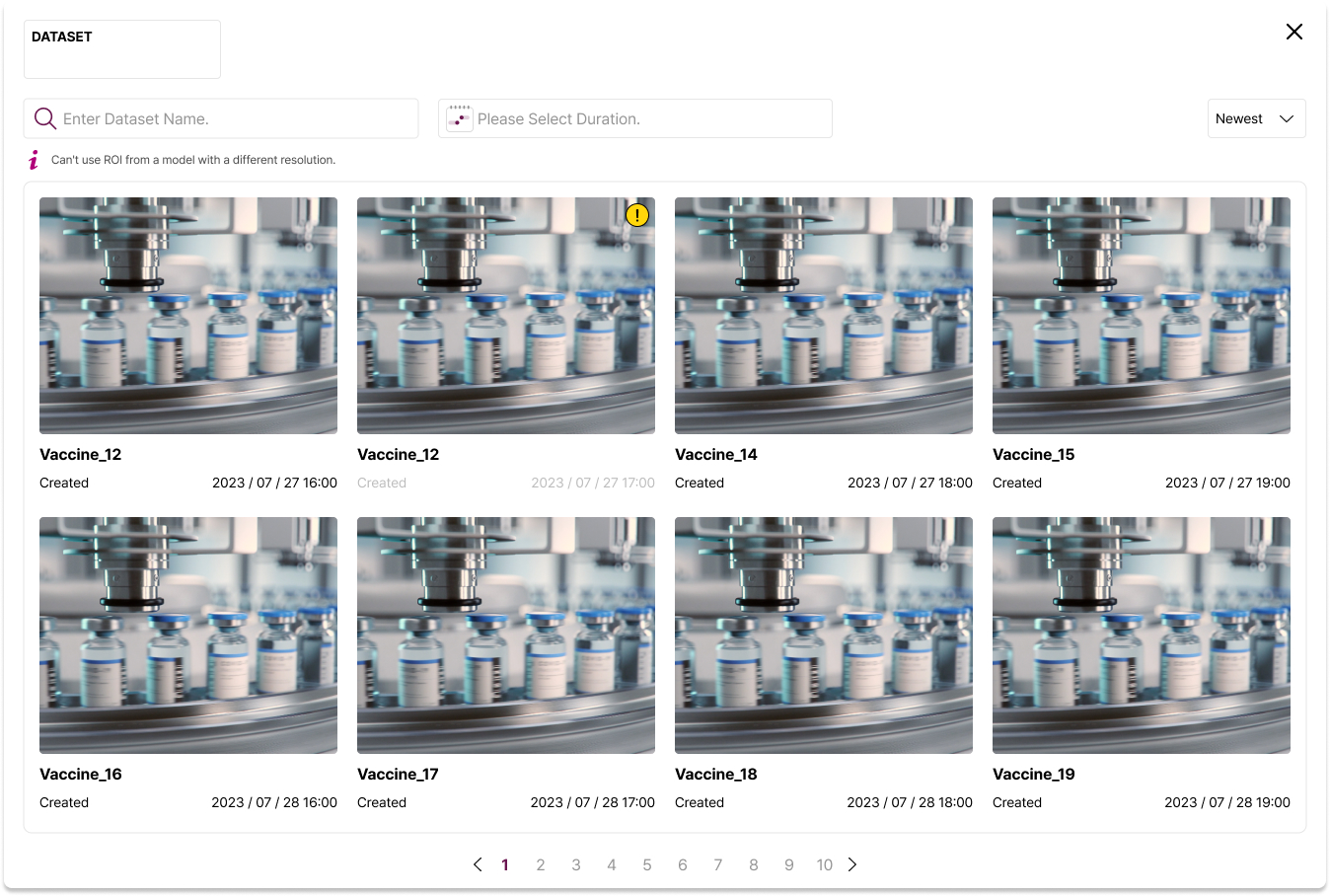
Model versioning/experiment reproduction/retraining
LISA provides model versioning capabilities. Track when and on what dataset each individual model was trained, and what learning presets were applied. Because all the information needed to verify the performance of a specific model is recorded, different users can reproduce the experiment under the same conditions.
It also offers the capability to retrain a specific model. Users can input additional datasets without reconstructing a model from scratch. By applying proven parameters, such as existing ROI and learning presets, users can maintain consistency and improve the model more quickly. Model development time can be significantly reduced, and new data can be quickly reflected in existing models to effectively respond to changing market requirements.
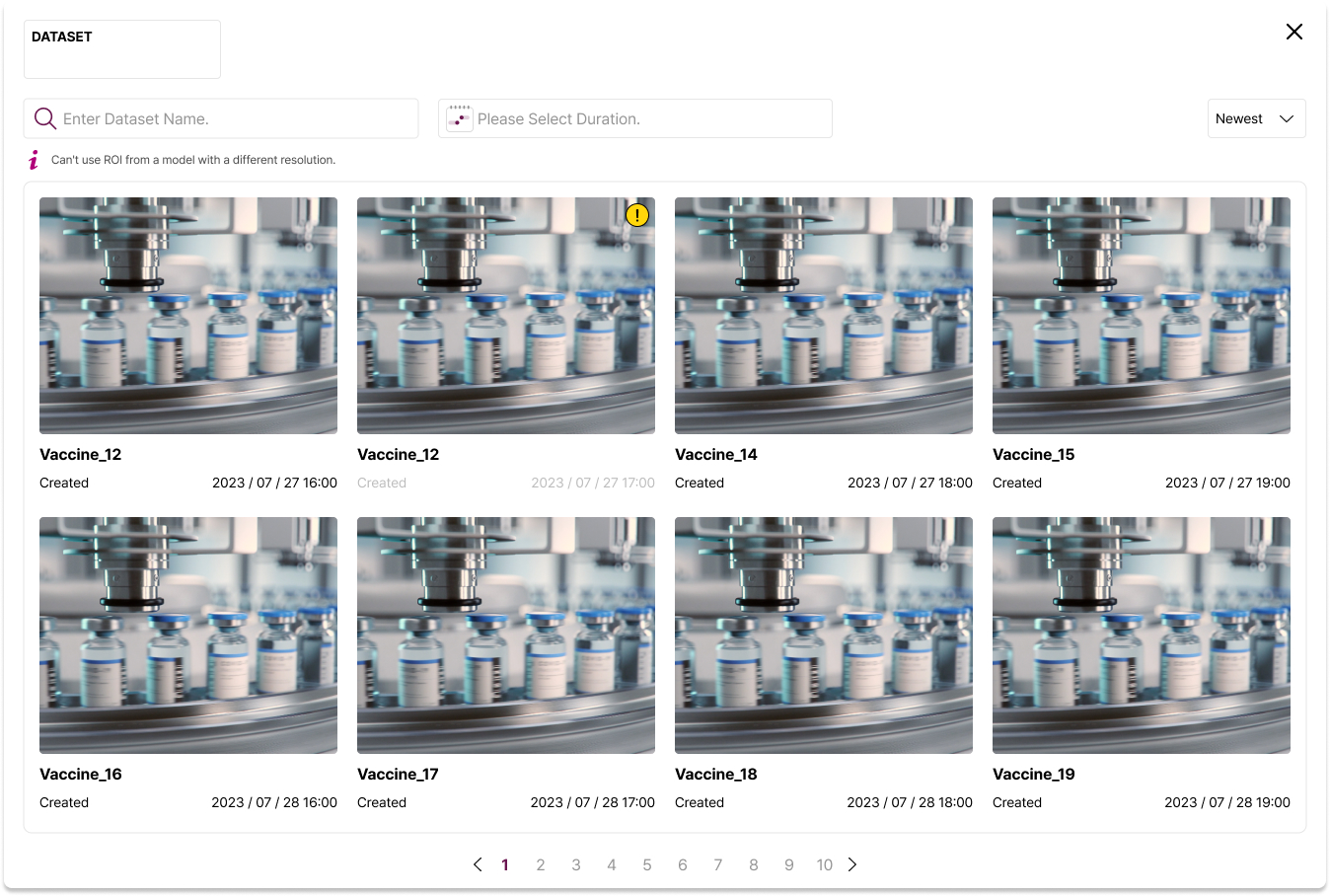
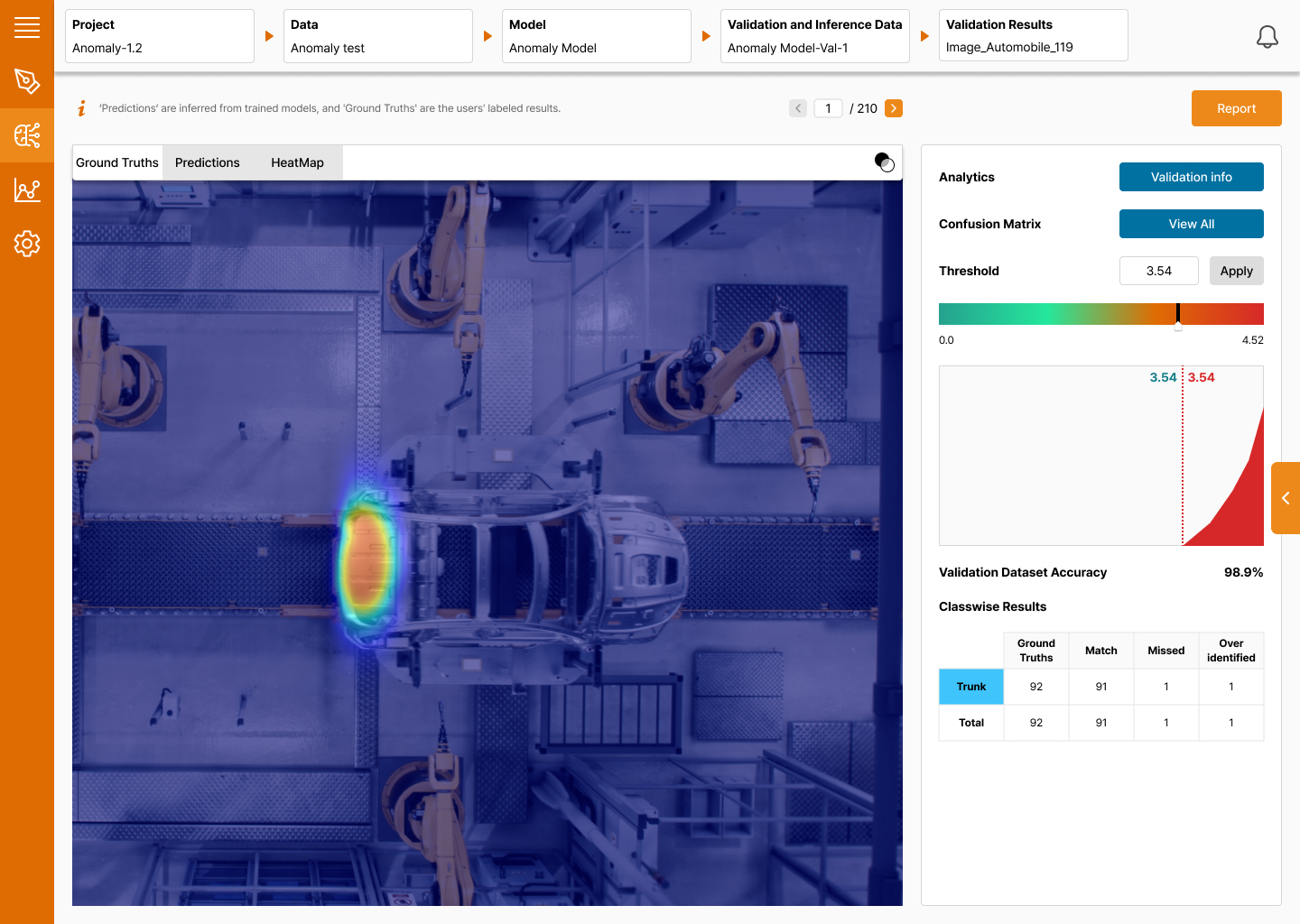
Improve model accuracy through easy threshold adjustment
LISA offers a visualized UI to easily identify anomaly detection sections during evaluation and testing, and supports easy adjustment of the model’s anomaly detection threshold after performance review. Users can instantly check results for various thresholds by dragging the inspection baseline up or down. If more precise control is needed, the user can directly enter the threshold number. In this way, by directly adjusting and experimenting with the sensitivity of the model, users can quickly achieve optimal model performance for a specific environment.
Free customization even if inspection standards change after field application
Inspection standards can be flexibly adjusted even after field application. Unlike competitor deep learning inspection models that require new data labeling and retraining when inspection rules change, users can easily adjust the threshold by moving the inspection baseline or directly entering a number. The system updates and displays anomaly detection results according to the changes in real time. This allows users to immediately evaluate the impact of threshold changes on real-world operations and find optimal settings, saving time and money on regenerating and deploying models.
Achieve Your Business Goals
with a Convenient MLOps Platform
Achieve Your Business Goals with a Convenient MLOps Platform
Detecting Inspection Data Drift Caused by Material Changes: A Case Study on the Data Quality Index (DQI) Model
Challenge In manufacturing, consistently capturing high-quality...
Predicting Motor Failures with Vibration Sensor Data (Predictive Maintenance)
Challenge Previously, vibration sensors were installed on power plant...
A successful case of reducing ‘false-positives’ by applying a deep learning model to detect ‘type 1 errors’
Challenge If the test standard is set too sensitively to achieve 0%...
Next Step →
Experience LISA, which is already achieving industry-leading speed and performance at global manufacturing sites.