Success case of monitoring ‘data drift’ and performing predictive maintenance with a data quality index (DQI) model
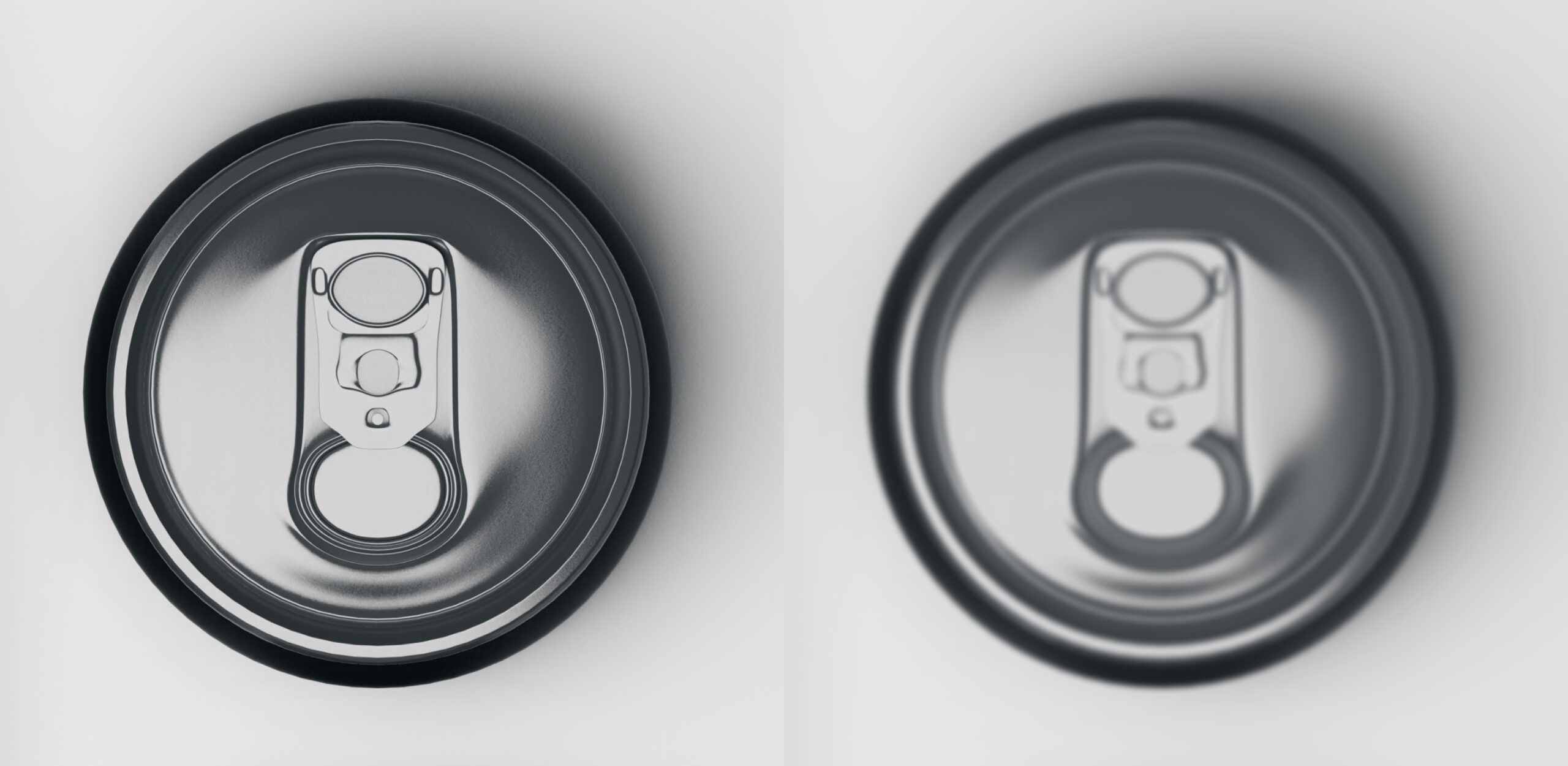
Challenge
- Consistent optical images must be taken at all times to properly train the model and increase the accuracy of quality control
- However, if the camera angle shifts or the lighting deteriorates, causing improper image capture, defect inspection fails
- Adding additional sensors or equipment to monitor the equipment can be costly and complex to manage
Approach
- We, the AHHA Labs team, propose a deep learning model (Data Quality Index (DQI)) that analyzes device data in addition to inspection data
- By using a normal image with a DQI score of 0 (baseline) for training, the deep learning model deduces the DQI score for abnormal images
- Alarms are triggered when the DQI score exceeds a certain threshold, allowing proactive responses before inspection failures accumulate
Result
- Monitoring “data drift” becomes possible
- Helps keep projects on schedule by eliminating unpredictable sources of failure early on
Full Story
No way to know in advance what’s wrong with your inspection equipment
There are various inspection equipment in a smart factory. The most representative is machine vision equipment, i.e., cameras and lighting, which requires constant optical images to properly train machine learning models and detect surface defects with high accuracy.
Sometimes, even if the product has no defects, it can still be marked NG for a period. This is often due to problems with the inspection equipment.
For example, during equipment cleaning or inspection, the camera might be bumped and reinstalled at a different angle, resulting in it being out of focus.
Or, as the lights age, the light level changes and the image is too dark. Or, less frequently, a worker in white walks by and the light reflects off of them, blowing the image white.
If the NG continues to occur due to an inspection equipment issue rather than a product defect, we have to stop the production line and find the cause, which can mean hours of downtime. If the problem is old lights, it can take days to order, receive, and re-install new lights. Not only that, but you’ll have to re-inspect the parts that were labeled NG because of image issues.
While adding sensors or equipment to monitor the inspection equipment was an option, the cost and management complexity were too high to ensure a return on investment (ROI).
Alerts when image quality starts to deteriorate
Company C, a manufacturer of rechargeable batteries for electric vehicles, approached AHHA Labs about using our Data CAMP solution to collect and analyze inspection image data.
During a custom consultation, we discovered that the customer had a pain point similar to the one described above. At the time, Data CAMP did not have a separate feature to analyze device data. The AHHA Labs team began to think about how they could identify inspection equipment anomalies using the Data CAMP solution alone, without the need for additional sensor attachments, and this is where the deep learning model called the Data Quality Index was born.
DQI works like this.
First, we train the model on normal images. When an abnormal image is introduced, the model infers how far the focus or brightness deviates from the normal image.
For example, a DQI score of 0.1, 0.2, 0.3… The further away from 0 the image is, the more blurred it is compared to normal. The same goes for brightness, location, and angle. As soon as an anomal alarm is raised, field operators can check their cameras and lights and react proactively.
Highlights
- Monitoring “data drift” becomes possible
- Helps keep projects on schedule by eliminating unpredictable sources of failure early on
Of course, it’s not every day that you encounter back-to-back NGs due to installation angle issues or failure of inspection equipment, but every time it happens, the losses are significant. As mentioned earlier, it takes hours to days to identify and fix the problem.
This results in downtime and is a significant source of production inefficiency. On top of that, all of the products that have been labeled NG due to bad images have to be re-inspected.
Company C now utilizes Data CAMP and the DQI model to achieve a 99.9% inspection success rate. This is because they monitor drift* in the images they capture, or input data, and are able to react to NGs after they have already occurred, whereas they can now proactively maintain their equipment using DQI scores.
Data drift is a change in the distribution of data over time. This can cause models that were accurate when trained to become less accurate over time after deployment. This phenomenon occurs when the statistical properties of the input data change, which can be caused by a variety of factors such as changing customer behavior, seasonal influences, economic changes, or simply the dynamic nature of the environment the model is applied to.
There was no additional cost or time invested here. We didn’t install any additional equipment or sensors, just machine learning algorithms.
AI Inspection with 86% Cost Reduction Using On-Device AI
Challenge Defects caused by external contaminants are unpredictable in shape,...
Transforming Battery Manufacturing with AI Inspection & Data Automation
From Manual Inspections to Smart Factories: The Evolution of Battery...
A 3-Step AI Inspection Pipeline for Rubber Sheet Defects: Automating Detection, Classification, and Location Analysis
Challenge In the tire manufacturing process, rubber sheets produced during...
Boosting Control Efficiency of Battery Assembly Lines by 300% Through a Remote Monitoring System
Challenge The assembly lines for EV batteries are massive and highly complex,...
Detecting Inspection Data Drift Caused by Material Changes: A Case Study on the Data Quality Index (DQI) Model
Challenge In manufacturing, consistently capturing high-quality...