Navigating the Future: Unleashing the Potential of Digital Twin Technology in Manufacturing
2023-11-21

The concept of Digital Twin has recently emerged as a core technological trend in the manufacturing industry. As the product life cycle becomes shorter and predicting product demand becomes more challenging, coupled with the increasing demand for customized products, the adoption of Digital Twin is being touted as a decisive factor for the survival of companies. What exactly is the concept of Digital Twin, and how can it be utilized in the manufacturing industry?
Concept of Digital Twin
Digital Twin is a convergent technology that creates a virtual replica (twin) of real-world objects in a virtual space, synchronizing it with real-world data. [1] Utilizing this technology, analysis and simulations can be performed to address real-world issues. Vehicle navigation systems are a common example of a Digital Twin in everyday life. By synchronizing real-world data, such as real-time traffic conditions, with a virtual road network, simulations can be conducted to find the optimal route to a destination.
In the manufacturing industry, digital models of machines, processes, and systems are commonly referred to as Digital Twins, which also is ‘navigation system for factories.’ In recent years, with significant advancements in technologies such as the Internet of Things, artificial intelligence, big data analytics, and virtual reality, the usage of Digital Twins for prediction, prevention, optimization, and other purposes in manufacturing has been on the rise.[2] According to Grand View Research in December 2022, the global market size in this field is predicted to grow at an average annual rate of 37.5%, reaching approximately $155.8 billion by 2030.[3]
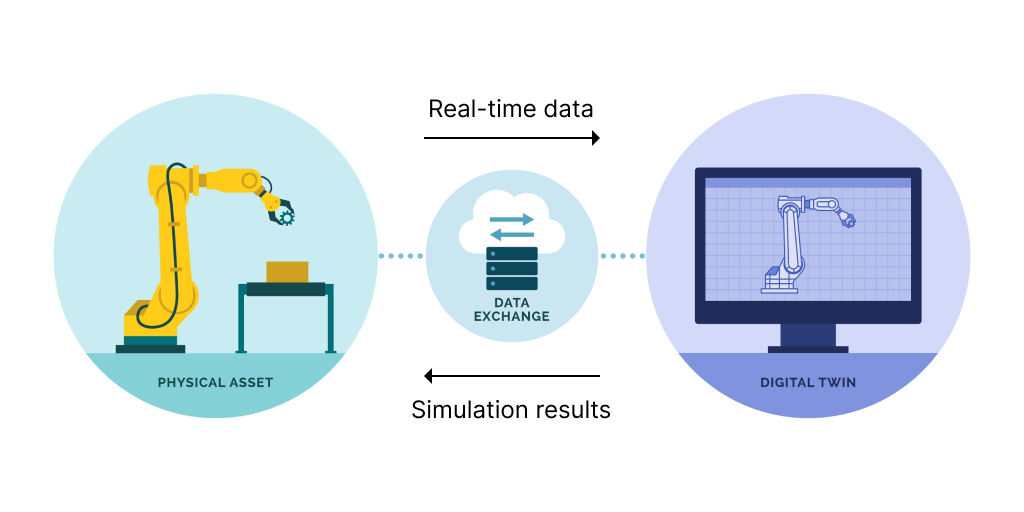
Digital Twin is a convergent technology that creates a virtual replica (twin) of real-world objects in a virtual space, synchronizing it with real-world data. Utilizing this technology, analysis and simulations can be performed to address real-world issues. – Image Credit : AHHA Labs
Five Stages of Advancement in Digital Twin Technology
Digital Twin technology consists of virtualization, synchronization, modeling and simulation, and federated digital twin technologies. The evolution is anticipated to progress through the stages of 1) Mirroring → 2) Monitoring → 3) Modeling & Simulation → 4) Federated and finally, 5) Autonomous.
Stage 1: Mirroring
This stage involves replicating and visualizing the physical object into a Digital Twin. It is unnecessary to recreate the real-world appearance perfectly; instead, the key is to use the Digital Twin to create the most suitable twin for the intended purpose.
A vehicle navigation system is a common example of a Digital Twin in everyday life. In the navigation map (twin), details like the thickness of the median strip or the height of surrounding buildings are not depicted. This is because these physical quantities are unnecessary for finding the fastest optimal route. Similarly, when implementing a Digital Twin in a manufacturing setting, it is crucial to clearly define the problem to be addressed and replicate it accordingly.
Recently, there has been a trend towards real-time 3D space implementation technology. Unity, a company known for game engines, has shifted its focus to visualize data in industrial environments. They have introduced a real-time 3D development platform, allowing developers to scan 3D spaces and extract information such as the terrain, buildings, and objects in that space. This extracted information can be utilized for various purposes, including creating Digital Twins or developing AR and VR content.

Real-time 3D Technology – Image Credit : Unity
Stage 2: Monitoring
This stage involves monitoring and controlling physical objects based on Digital Twins. For instance, navigation systems reflect real-time traffic conditions and accident updates, not just the GPS information of your vehicle.
In this phase, synchronous technology that allows real-time reception of real-world information is essential. The advancement of sensor and IoT (Internet of Things) technologies, coupled with the refinement of data collection, preprocessing, and analysis technologies, drives the progression of Digital Twin development.
Recently, with the development of artificial intelligence (AI) technology, industrial AI has been actively researched and adopted for control purposes. For example, the use of AI in ‘Situation Awareness’ : in this case, Anomaly Detection[6] deep learning algorithms are integrated into CCTV systems, so it can detect abnormal situations in manufacturing environments, such as a fire outbreak, equipment malfunctions, deviations from planned production leading to defective products, and send alerts to users.
Stage 3: Modeling & Simulation
The goal of implementing Digital Twin is to solve complex real-world problems. Therefore, the simulation function must be seamlessly integrated.
Over many years, simulations have been widely used in various fields. The key distinction between Digital Twin and traditional simulations lies in whether real-world data is synchronized in real-time. While traditional simulations made predictions about performance at a certain future point by assuming various surrounding situations and physical quantities, Digital Twin actively seeks optimal solutions by continuously reflecting real-time changes in surrounding physical quantities. This leads to achieving higher accuracy in a prediction.
Recent remarkable advancements in reinforcement learning [7] deep learning algorithms, the development of big data collection and analysis technologies, and improvements in computing performance make faster and more extensive simulations possible. For instance, in the manufacturing industry, Digital Twins are employed to simulate and identify design issues on blueprints before the actual construction of a new factory.
Simulations can also be utilized to optimize the design of new products. In practice, companies like Yaskawa Electric Corporation and JSR Corporation in Japan succeeded in autonomously operating a chemical plant for 35 days using AI based on reinforcement learning in 2022.[8] It was possible because the AI discovered complex conditions to maintain the liquid level in the distillation column appropriately while maximizing the utilization of waste heat as a heat source. As a result, they achieved ideal results in quality stabilization, high productivity and energy savings.
Effortlessly conduct the data collection and analysis needed for implementing Digital Twins with AHHA Labs’ innovative solutions.
Stage 4: Federated
This stage involves integrating two or more optimized individual Digital Twins into interconnected Twins. In recent academic research, there has been an active exploration of Federated Twins.
Currently, Digital Twins predominantly focuses on optimizing individual objects, processes, or systems (facilities). In the future, Digital Twins will be used in various industrial domains. These multiple digital twins will collaborate to address complex problems, considering various causative factors.[2] Product manufacturing involves combining the digital twins of the factory, logistics vehicles, and the entire city to monitor the expanded product lifecycle from raw material supply to product disposal. This enables the identification of the most optimized production methods.
Stage 5: Autonomous
This stage involves a fully autonomous operation where a single or federated Digital Twin autonomously identifies issues and implements solutions. While Smart Factories [9] up to now have operated automatically within predefined human-set parameters, in the future, autonomous Digital Twins will be able to predict equipment failures in advance, optimize processes on their own, and autonomously control unforeseen issues.
Examples of Manufacturing Innovation Using Digital Twins
(1) Design Optimization
German global automotive company BMW is already producing cars in its digital twin of the Debrecen plant in Hungary, which is set to start manufacturing in 2025. The company established a digital twin of over 7 square kilometers of indoor space and 150 square kilometers of outdoor production space by 3D scanning of the vehicle and engine factories.
BMW utilizes NVIDIA’s Omniverse AI system in this virtual factory to perform simulations. For instance, using the digital twin, they visually verify production simulations and optimize the flow of assembly lines. Changing existing equipment or flows incurs significant additional costs and causes downtime, but BMW smartly avoids them by pre-optimizing them using digital twins.[12]
Through this process, BMW simulated all elements of the entire factory, including production personnel, robots, and assembly components, thereby shortening the planning time, and expecting to improve production efficiency by over 30%.[13] Furthermore, they aim to leverage digital twins to reduce the planning process for a new production site, which previously took three years, to two years.
Boeing, a giant aerospace company, utilizes digital twins for aircraft design. After creating a digital twin for a new aircraft, they run simulations predicting the performance of various elements that constitute the aircraft throughout its product lifecycle. As a result, Boeing engineers can predict when the newly designed aircraft might experience failures. According to Boeing, the initial improvement rate in component quality through digital twins reached 40%. Boeing has set a goal to digitize all development systems in the near future.
(2) Operational Optimization
Operational testing, which verifies whether the facilities operate correctly, and the products are accurately produced, is crucial in manufacturing. POSCO, a South Korean steelmaker, has established a ‘Digital Twin Steelworks’ to streamline its operations. By performing test operations in
the digital twin, they reduce the likelihood of potential issues in advance and cut related costs.
For instance, due to the ups and downs of raw material prices and carbon emission issues, steel mills must constantly adjust the input and blending of fuel and raw materials. In the past, considering numerous components of raw materials and comprehensively verifying their impact on the process required significant manpower and time. Now, before inputting fuel or raw materials, the Digital Twin Steelworks conducts simulations to find the optimal blend at the minimum cost.[14] Using digital twins, they can simulate over 125,000 scenarios in the steelmaking process, increasing the temperature hit rate from 80% to 90%. The ‘Digital Twin Steelworks’ affects various outcomes that can impact quality, cost, production, and predicts carbon dioxide emissions changes from an ESG perspective within two minutes.
Dutch multinational consumer goods company Unilever provides a leading example of how general manufacturing companies can adopt digital twins. They optimize production conditions with a digital twin at their laundry detergent powder factory in Indaiatuba, Brazil. Through this, Unilever has reduced the number of alarms requiring daily attention by up to 90%, minimizing process interruptions and production time. This allows employees to intervene in a timely manner, enhancing operational efficiency.
In April 2023, Unilever collaborated with IT companies Accenture and Microsoft to overhaul existing on-premises systems into a cloud platform. This move provided a more stable foundation for using digital twins, accelerating decision-making. Unilever aims to expand digital twins to connect 300 factories worldwide.[15]
(3) Fault Prediction
Hyundai Motor, a South Korean automotive company, conducts technical pre-verification using digital twins to manage the performance of electric vehicle batteries.[16] Hyundai’s idea is that a digital twin synchronizes various driving data collected from electric cars on the road to a virtual digital electric vehicle to predict the battery life. Using machine learning algorithms, they aim to know more accurate battery life for each vehicle by comprehensively analyzing vehicle-specific information such as charging/discharging, driving habits, parking and driving environment, which can impact battery performance.
In the future, Hyundai plans to use this technology to assist customers in optimizing battery performance. They aim to implement technologies that provide tailored advice to users, such as ‘try using slow charging when you have time,’ ‘speeding affects battery life,’ and ‘ventilated seats are more energy-efficient than continuous use of the air conditioner.’
Conclusion | Innovation Starting from Data Collection
In the manufacturing industry, digital twins hold diverse potential. However, there are still not many cases because of the lack of convincing success stories, which ensure the cost and effort to adopt digital twin systems.
According to the Smart Manufacturing Innovation Promotion Team affiliated with the Korea Institute of Industrial Technology Information (KITECH), as of 2023, 74.7% of manufacturing companies are currently at the basic level of smart factories.[17] The basic level refers to the extent of automatically aggregating production performance information or real-time understanding of logistics flows. When translated into the stage of digital twin advancement, it is within partial implementations of stage 1 (simulation) and 2 (control).
While many manufacturing companies perceive digital twins as fully autonomous factories (stage 5) and feel overwhelmed, implementing stage 5 digital twins from the beginning is impossible.
Digital Twin can be started quickly by collecting data generated in various manufacturing processes, quality management systems, raw material warehouses, and other locations on the factory floor. When all data is integrated and monitored collectively, visibility into the entire process increases, leading to significant efficiency improvements. Accumulating results also allows for real-time decision-making and control of the process system. After the application of this stage, digital twin systems can perform simulations to address real-world problems, such as identifying the causes of defects or deriving optimal conditions for high productivity. By taking these steps, you will finally achieve your goals to successfully introduce a perfect-fitted digital twin system for your manufacturing site.
Effortlessly conduct the data collection and analysis needed for implementing Digital Twins with AHHA Labs’ innovative solutions.
Chloe Woo | Content Strategist
Reference
- https://www.yna.co.kr/view/AKR20221123025501017
- https://www.dbpia.co.kr/journal/articleDetail?nodeId=NODE11227543
- Grand View Research
- https://mobile.newsis.com/view.html?ar_id=NISX20231005_0002472776#_PA
- Unity
- Anomaly Detection Wikipedia
- Reinforcement Learning Wikipedia
- https://www.aitimes.kr/news/articleView.html?idxno=24598
- Smart Manufacturing Wikipedia
- https://ksp.etri.re.kr/ksp/plan-report/read?id=996
- https://mobile.newsis.com/view.html?ar_id=NISX20220817_0001980727
- https://www.madtimes.org/news/articleView.html?idxno=17171
- https://biz.chosun.com/site/data/html_dir/2021/04/19/2021041901953.html
- https://newsroom.posco.com/kr/
- Iot One
- https://www.hyundai.co.kr/news/CONT0000000000032186
- https://www.smart-factory.kr/usr/pr/sf/ma/smrtFctryIntrcn
Related Stories
AHHA Labs Showcased Its Solutions at the Plug and Play 2024 Silicon Valley Summit!
From November 19 to 21 (local time), AHHA Labs participated in the Plug and Play 2024 Silicon Valley Summit, showcasing its solutions on a global stage! Plug and Play (PNP), headquartered in Silicon Valley, is the world’s largest accelerator, having nurtured...
A successful case of reducing ‘false-positives’ by applying a deep learning model to detect ‘type 1 errors’
Challenge If the test standard is set too sensitively to achieve 0% ‘false-negative’, ‘false-positive (type 1 error)’ increases. Inefficiency due to random sampling and visual inspection to detect false-positives Approach LISA's type 1 error inference...
Success case of monitoring ‘data drift’ and performing predictive maintenance with a data quality index (DQI) model
Challenge Consistent optical images must be taken at all times to properly train the model and increase the accuracy of quality control However, if the camera angle shifts or the lighting deteriorates, causing improper image capture, defect inspection fails...
Configuring an AI model pipeline to detect robotic grasping anomalies in real time
Challenge Robot drops battery, causing downtime Difficulty utilizing existing machine learning solutions due to 'class imbalance' problem Difficulty treating footage with no battery for the robot to grab in the first place as separate normal data Approach...
How the Anomaly Detector model was used to automate the quality control process using only normal data
Challenge Pouch-type batteries have flexible surface geometry, complicating defect identification. This makes it challenging to use existing rule-based inspections and deep learning model-based inspections like classification and segmentation Finding skilled...