Mastering Real-Time Anomaly Detection with LISA: Your Gateway to Industrial Excellence
11/01/2023
As the global manufacturing industry accelerates its transition towards smart factories, there is a growing interest in automated inspection, real-time anomaly detection, and predictive maintenance through machine vision. This interest is particularly pronounced in innovative manufacturing sectors like semiconductors and secondary batteries, where thousands of components and products are produced daily. The manual identification of defects by humans has become unfeasible in such high-production environments. Today, customers are unforgiving even of subtle defects, making the quick and accurate identification of defects through AI inspection automation essential to meet this demand.
AHHA Labs’ LISA (Look In Smart with AI) is an AI solution designed for managing manufacturing environments and ensuring product quality. By leveraging machine learning on a diverse range of data collected from industrial sites, LISA can identify real-time abnormalities, and its unique methodology enhances the precision of deep learning models.
LISA enables the effortless detection of irregularities in the smart factory’s manufacturing processes by analyzing CCTV images. It also allows for the supervision of product quality metrics via AI-driven vision inspections and the evaluation of real-time conditions and anomalies by analyzing time-series data gathered from instrumentation sensors.
How LISA Automates Inspections
1. Unsupervised Anomaly Detection : Training with a Minimal Amount of Normal Data Only
LISA can train a deep learning model even when there is no data available for abnormal samples, meaning product defects. Take a closer look at LISA’s unique two-step approach for rapidly improving model accuracy:
Step 1: Unsupervised Learning through Normal Data → Initial Classification of Anomalies
Typically, various deep learning algorithms, such as classification, object detection, and segmentation, are used in AI solutions. By training the deep learning model with both normal and abnormal samples, it becomes capable of detecting process abnormalities or product defects.
Object Recognition Deep Learning Algorithms:
- Classification: Identifying the class of an object (normal or abnormal, cat or dog).
- Object Detection: Locating the positions of multiple objects and then classifying them using bounding boxes.
- Segmentation: Identifying the actual edges of multiple objects and classifying them, without using bounding boxes.
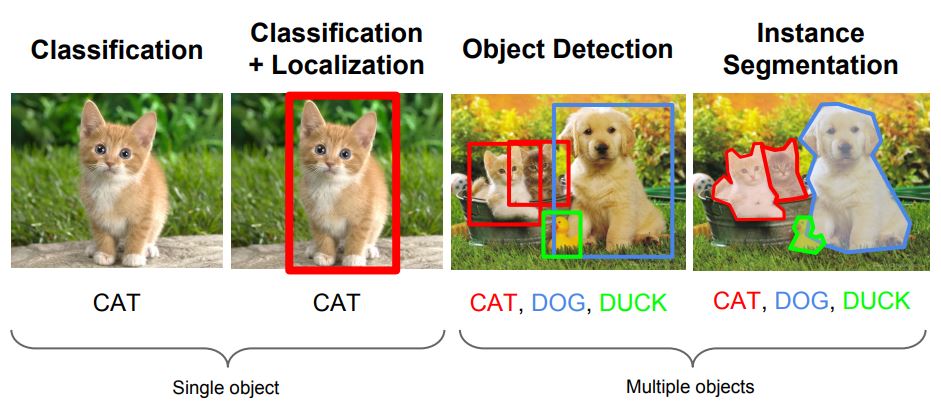
Image Credit : Fei-Fei Li, Andrej Karpathy & Justin Johnson (2016) cs231n, Lecture 8 – Slide 8, Spatial Localization and Detection
However, in industrial settings, a challenge known as ‘class imbalance’ arises. To create a high-performance AI model by training the deep learning algorithms mentioned earlier in a balanced manner, it is essential to have an equal mix of normal and abnormal data. In practical industrial environments, however, the amount of abnormal data is typically much lower than that of normal data. This is understandable, as an excessive number of defective products would render factory operations impractical. For instance, if 20,000 normal products are produced, while only one defective product is produced on average, you would need to manufacture a million products to obtain a mere 50 abnormal data points. Especially when establishing new factories or introducing new processes using the latest technology, acquiring sufficient data can be highly time-consuming and costly.
In such situations, Anomaly Detection algorithms can offer an excellent solution. These algorithms allow you to perform *unsupervised learning using only normal data. The core concept involves defining the boundaries for the ‘normal’ category narrowly and classifying anything outside of these boundaries as ‘abnormal.’ For example, by quickly producing and training on just 100 videos or images of normal processes or products, you can effectively identify any deviations from this pattern.
- Unsupervised Learning is a method of training on unlabeled data without performing a pre-labeling process to determine the clusters or categories to which the data belongs, such as normal or abnormal.
LISA employs all four methodologies to detect defects with a high level of sensitivity. Furthermore, by utilizing anomaly detection algorithms, it can discover entirely new types of defects that were previously unknown. In contrast to the other deep learning algorithms mentioned earlier (classification, object detection, segmentation, etc.), which require the creation of abnormal samples for every defect case, they can only identify defects that are already known.
Step 2: User Feedback → Secondary Abnormal Data Classification
Now, users review the abnormal data initially filtered by LISA and label it, determining whether it genuinely represents a defect or if it’s a ‘false positive,’ mistakenly classified as defective when it is not. By training the model with this labeled data, the model’s accuracy can be further improved.
2. Zero False Negatives, Real-Time Processing Performance with Low-Latency
LISA’s deep learning model achieves a 0% rate of ‘missed'(false negative) cases, meaning it never fails to detect actual defects. For instance, in the case of secondary batteries used in electric vehicles, maintaining a high level of detection sensitivity is crucial, as failing to identify a defect within the manufacturing process could lead to severe safety risks and substantial financial losses.
Reducing ‘false positive’ cases, in which a non-defective item is mistakenly classified as defective, can be achieved rapidly. LISA’s two-step approach, combining unsupervised learning and user feedback, allows for optimizing both ‘missed’ and ‘false positive’ cases, approaching a rate of 0.
LISA enables real-time processing of 30 frames of video without any delay. When using only classification, object detection, and segmentation algorithms for optical image processing, it maintains a tact time of 10 ms per product based on a 2-megapixel image resolution. Even during prolonged operation, the processing time remains consistent.
3. User-Friendly Approach
LISA is designed with a user-friendly UI/UX suitable for general users, making it intuitive to manage data and models. This advantage means that anyone, even those without expertise in AI, can easily use it.
Engineers responsible for manufacturing processes are experts in their field, but they might find industrial AI to be unfamiliar territory. Such a knowledge gap can often be a significant barrier to the digital transformation of smart factories. To enhance smart factories, collaboration between process experts, big data specialists, and AI experts is ideal, but the associated costs and time constraints can be prohibitive.
Leveraging LISA enables individuals to effortlessly navigate the entire workflow, spanning data acquisition via video or image recording, defining learning parameters, model selection, parameter configuration, model creation, data training, evaluation, and real-time anomaly detection, all guided by a straightforward manual reference.

LISA’s User Interface. Designed with User-Friendly UI/UX for Easy Data and Model Management: Accessible to Anyone, Even without AI Expertise. Image credit : AHHA Labs
4. Enhancing Collaboration with LISA: Instant Access for Remote Teams
LISA operates in a web-based environment, making it highly accessible without significant effort.
Traditional Windows-based applications are limited to on-site use. To provide access to multiple workers from various locations, the easiest solution is to employ remote desktop connections.
However, remote connections come with security vulnerabilities. When multiple users connect remotely, there is a higher risk of affecting the main service. For example, if someone accidentally closes an inspection window, it could disrupt the inspection process.
LISA, on the other hand, allows multiple users to share information about training progress, real-time anomaly detection, and more with just simple profile configurations. For instance, an engineer working at a headquarters office far from the factory can access real-time anomaly detection results by connecting to the server. As it operates as a server-client setup, users accessing the server do not impact the main service.